مقاله انگلیسی رایگان در مورد تحلیل تصویر اشعه ایکس برای شناسایی پوکی استخوان – الزویر ۲۰۲۳
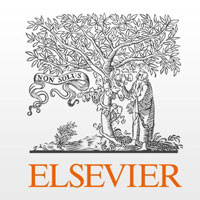
مشخصات مقاله | |
ترجمه عنوان مقاله | تجزیه و تحلیل تصویر اشعه ایکس برای تشخیص پوکی استخوان: از تجزیه و تحلیل کم عمق تا عمیق |
عنوان انگلیسی مقاله | X-ray image analysis for osteoporosis diagnosis: From shallow to deep analysis |
نشریه | الزویر |
انتشار | مقاله سال ۲۰۲۳ |
تعداد صفحات مقاله انگلیسی | ۱۵ صفحه |
هزینه | دانلود مقاله انگلیسی رایگان میباشد. |
نوع نگارش مقاله |
مقاله پژوهشی (Research Article) |
مقاله بیس | این مقاله بیس میباشد |
نمایه (index) | Scopus – Master Journal List – JCR |
نوع مقاله | ISI |
فرمت مقاله انگلیسی | |
ایمپکت فاکتور(IF) |
۳٫۳۳۳ در سال ۲۰۲۰ |
شاخص H_index | ۵۹ در سال ۲۰۲۲ |
شاخص SJR | ۰٫۳۹۳ در سال ۲۰۲۰ |
شناسه ISSN | ۰۱۴۱-۹۳۸۲ |
شاخص Quartile (چارک) | Q2 در سال ۲۰۲۰ |
فرضیه | ندارد |
مدل مفهومی | دارد |
پرسشنامه | ندارد |
متغیر | دارد |
رفرنس | دارد |
رشته های مرتبط | پزشکی |
گرایش های مرتبط | ارتوپدی |
نوع ارائه مقاله |
ژورنال |
مجله | نمایش ها – Displays |
دانشگاه | Laboratoire de Génie Electrique (LABGET), Larbi Tebessi University, Algeria |
کلمات کلیدی | پوکی استخوان – استخراج ویژگی – تجزیه و تحلیل عمیق – فیلتر گابور – HOG – LPQ – الگوریتم خفاش |
کلمات کلیدی انگلیسی | Osteoporosis – Feature extraction – Deep analysis – Gabor filter – HOG – LPQ – Bat algorithm |
شناسه دیجیتال – doi |
https://doi.org/10.1016/j.displa.2022.102343 |
لینک سایت مرجع | https://www.sciencedirect.com/science/article/pii/S0141938222001615 |
کد محصول | e17312 |
وضعیت ترجمه مقاله | ترجمه آماده این مقاله موجود نمیباشد. میتوانید از طریق دکمه پایین سفارش دهید. |
دانلود رایگان مقاله | دانلود رایگان مقاله انگلیسی |
سفارش ترجمه این مقاله | سفارش ترجمه این مقاله |
فهرست مطالب مقاله: |
Abstract ۱ Introduction ۲ Osteoporosis overview ۳ Related works ۴ Material and methods ۵ Results ۶ Discussion ۷ Conclusion Declaration of Competing Interest Acknowledgments Data availability References |
بخشی از متن مقاله: |
Abstract Recently, automated disease diagnosis based on medical images has become an integral component of digital pathology packages. Texture analysis is commonly used to address this issue, particularly in the context of estimating the osteoporosis progression in bone samples. Most research in this context uses handcrafted methods to directly extract bones image features despite the substantial correlation between sick and healthy bones, which explains the limited results. In this work, the handcrafted feature extraction method (e.g. HOG and/or LPQ) will be applied to a set of descriptors obtained from a deep analysis of bone texture images using Gabor’s filter bank. In addition, the classifier automatically adjusts the Gabor filters settings, using the bat-inspired algorithm based optimization, to achieve deep analysis behavior and optimal performance. Using a typically osteoporosis database, our experimental results reveal a significant improvement over the state-of-the-art deep/handcrafted techniques, resulting in an excellent performance of 89.66% for osteoporosis diagnosis. Introduction In 1895, Roentgen [1] may have been the first to notice that X-rays could be used to acquire an image of the organs inside the human body, from which one could determine whether or not there was disease. Since then, medical imaging using X-rays has become an important diagnostic tool for a variety of diseases. In fact, information extracted from images plays an important role in decision-making at many stages of patient care, including detection, characterization, staging, evaluation of treatment response, follow-up and evaluation of residual and recurrent disease after treatment, and surgery and radiotherapy guidance [2]. Despite the incredible development of medical imaging devices, the analysis and interpretation of captured images remains a major challenge for radiologists, because any error in interpretation can lead to the prescription of the wrong drugs, thus putting the patient in danger and destroying the success of the treatment. For example, on mammogram images, it can be difficult for radiologists, with a subjective examination, to distinguish between a tumor and a calcification. It is also difficult for them to detect with the naked eye the presence of a tumor in the dense breast [3]. Due to the inexperience of many radiologists and the large number of cases reviewed periodically, the treatment process will be expensive due to the large number of errors. So, to avoid or cut down on diagnostic mistakes, radiologists need a way to help them make the right decisions. Soft computing paradigms are a good way to do this. Conclusion Recent increases in the need for radiologists are primarily due to the rapid growth of medical imaging due to advancements in imaging technologies. Indeed, following the increase in demand, the workload of the radiologist has increased, which can unfortunately lead to diagnostic errors due to the specialists workload. Recently, the application of Artificial Intelligence (AI) approaches to the clinical practice of medical imaging has played a significant role in enhancing diagnostic accuracy and efficacy. In addition to improving diagnostic accuracy, these strategies bridge the gap between inexperienced and experienced clinicians or between generalists and specialists. The diagnosis of osteoporosis is one of the most crucial diagnostic practices requiring medical imaging. In this paper, we present an AI-based technique for automatically detecting osteoporosis by analyzing X-ray images of bone tissue. In this study, we developed a model for diagnosing osteoporosis based on handcrafted features taken from descriptors obtained from a thorough analysis of the bone image using a set of Gabor filters with different orientations. Two well-know hand-crafted methods were used to extract descriptor features, namely HOG and LPQ. To achieve a high level of performance, we used a bat-based optimization method to determine appropriate Gabor filter parameters. Additionally, we combined information at two distinct levels, including fusion at score level and fusion at decision level. In this study, we have attempted to cover all aspects of system development. Consequently, our studies consisted of selecting the optimal parameters of the normalization approach, deciding whether to analyze the full image or to analyze it in blocks, selecting the Gabor filter parameters, and lastly combining the information from various subsystems. In fact, all of these tests resulted in an excellent performance (ACC = 89.66%) that outperformed several previously published studies. In future work, we intend to combine these features with clinical data to develop a multimodality model. In addition, we will try to use/develop more predictive handcrafted features to improve the accuracy of the diagnosis. |