مقاله انگلیسی رایگان در مورد رویکرد فشرده سازی اطلاعات اینترنت اشیا – الزویر ۲۰۱۹
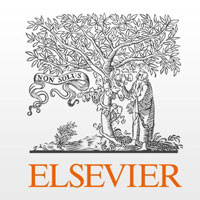
مشخصات مقاله | |
ترجمه عنوان مقاله | یک رویکرد فشرده سازی اطلاعات اینترنت اشیای موثر انرژی در یادگیری ماشین لبه ای |
عنوان انگلیسی مقاله | An energy efficient IoT data compression approach for edge machine learning |
انتشار | مقاله سال ۲۰۱۹ |
تعداد صفحات مقاله انگلیسی | ۳۰ صفحه |
هزینه | دانلود مقاله انگلیسی رایگان میباشد. |
پایگاه داده | نشریه الزویر |
نوع نگارش مقاله |
مقاله پژوهشی (Research Article) |
مقاله بیس | این مقاله بیس نمیباشد |
نمایه (index) | Scopus – Master Journals List – JCR |
نوع مقاله | ISI |
فرمت مقاله انگلیسی | |
ایمپکت فاکتور(IF) |
۷٫۰۰۷ در سال ۲۰۱۸ |
شاخص H_index | ۹۳ در سال ۲۰۱۹ |
شاخص SJR | ۰٫۸۳۵ در سال ۲۰۱۸ |
شناسه ISSN | ۰۱۶۷-۷۳۹X |
شاخص Quartile (چارک) | Q1 در سال ۲۰۱۸ |
رشته های مرتبط | مهندسی کامپیوتر، فناوری اطلاعات |
گرایش های مرتبط | هوش مصنوعی، اینترنت و شبکه های گسترده |
نوع ارائه مقاله |
ژورنال |
مجله / کنفرانس | سیستم های کامپیوتری نسل آینده-Future Generation Computer Systems |
دانشگاه | FEMTO-ST Institute, UMR 6174 CNRS, Univ. Bourgogne Franche-Comt´e, France |
کلمات کلیدی | اینترنت اشیا، رایانش لبه ای، فشرده سازی اطلاعات، یادگیری ماشین، راندمان انرژی، تشخیص تنش |
کلمات کلیدی انگلیسی | IoT, Edge computing, Data compression, Machine learning, Energy efficiency, Stress detection |
شناسه دیجیتال – doi |
https://doi.org/10.1016/j.future.2019.02.005 |
کد محصول | E12068 |
وضعیت ترجمه مقاله | ترجمه آماده این مقاله موجود نمیباشد. میتوانید از طریق دکمه پایین سفارش دهید. |
دانلود رایگان مقاله | دانلود رایگان مقاله انگلیسی |
سفارش ترجمه این مقاله | سفارش ترجمه این مقاله |
فهرست مطالب مقاله: |
Abstract ۱٫ Introduction ۲٫ Related work ۳٫ Error-bounded lossy compression ۴٫ Case study ۵٫ Experimental results and analysis ۶٫ Discussion ۷٫ Conclusion Acknowledgment References |
بخشی از متن مقاله: |
Abstract
Many IoT systems generate a huge and varied amount of data that need to be processed and responded to in a very short time. One of the major challenges is the high energy consumption due to the transmission of data to the cloud. Edge computing allows the workload to be offloaded from the cloud at a location closer to the source of data that need to be processed while saving time, improving privacy, and reducing network traffic. In this paper, we propose an energy efficient approach for IoT data collection and analysis. First of all, we apply a fast error-bounded lossy compressor on the collected data prior to transmission, that is considered to be the greatest consumer of energy in an IoT device. In a second phase, we rebuild the transmitted data on an edge node and process it using supervised deep learning techniques. To validate our approach, we consider the context of driving behavior monitoring in intelligent vehicle systems where vital signs data are collected from the driver using a Wireless Body Sensor Network (WBSN) and wearable devices and sent to an edge node for stress level detection. The experimentation results show that the amount of transmitted data has been reduced by up to 103 times without affecting the quality of medical data and driver stress level prediction accuracy. Introduction Cloud computing that is centrally deployed on a global scale has become an indispensable part of processing IoT data. However, cloud-assisted Internet of things (CoT) faces several difficulties such as transmission latency, bandwidth constraints, and high energy consumption. For instance, sending a single bit of data over the cellular network consumes a lot of energy which decreases the lifetime of the IoT system. On the other hand, edge computing has emerged as a promising paradigm that pushes the cloud services to the edge of the network. It can be seen as a decentralized cloud that drives the computing power closer to the source of data and allows local decision making. Edge computing was shown to be a better solution than the cloud in numerous IoT applications [1]. For instance, applications that demand near real-time responses such as autonomous driving cars and eHealth can not work properly with the cloud due to the high latency and ineffective bandwidth caused by the large number of sensors connected to the network. Wireless Sensor Networks (WSNs), Wireless Body Sensor Networks (WBSNs), and wearable devices make up the essential blocks of IoT architectures. Many of these smart objects, that are responsible for the collection, processing, and transmission of data, are still battery operated and resource constrained. The three major constituents of a smart object that consume energy are the microcontroller (MCU), transceiver, and sensor units. Among all tasks, it is well known that data transmission is the highest energy-consuming task in IoT nodes [2] [3]. An important step towards energy efficiency in IoT applications is the transfer of computational tasks from the cloud to the edge. In general, the radio communication task between the IoT nodes and the edge consumes less energy than transmitting the data directly to the cloud over the cellular network [1] [4]. |