مقاله انگلیسی رایگان در مورد پیش بینی رفتار مسافر با مدل حافظه کوتاه مدت طولانی – IEEE 2019
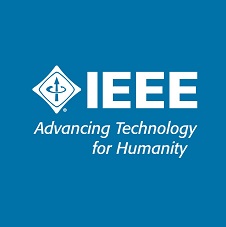
مشخصات مقاله | |
ترجمه عنوان مقاله | پیش بینی رفتار مسافر با مدل LSTM (حافظه کوتاه مدت طولانی) معنایی و چند الگویی |
عنوان انگلیسی مقاله | Passenger Behavior Prediction With Semantic and Multi-Pattern LSTM Model |
انتشار | مقاله سال ۲۰۱۹ |
تعداد صفحات مقاله انگلیسی | ۱۰ صفحه |
هزینه | دانلود مقاله انگلیسی رایگان میباشد. |
پایگاه داده | نشریه IEEE |
نوع نگارش مقاله |
مقاله پژوهشی (Research Article) |
مقاله بیس | این مقاله بیس نمیباشد |
نمایه (index) | Scopus – Master Journals List – JCR |
نوع مقاله | ISI |
فرمت مقاله انگلیسی | |
ایمپکت فاکتور(IF) |
۴٫۶۴۱ در سال ۲۰۱۸ |
شاخص H_index | ۵۶ در سال ۲۰۱۹ |
شاخص SJR | ۰٫۶۰۹ در سال ۲۰۱۸ |
شناسه ISSN | ۲۱۶۹-۳۵۳۶ |
شاخص Quartile (چارک) | Q2 در سال ۲۰۱۸ |
مدل مفهومی | ندارد |
پرسشنامه | ندارد |
متغیر | ندارد |
رفرنس | دارد |
رشته های مرتبط | مهندسی کامپیوتر، مهندسی عمران |
گرایش های مرتبط | مهندسی نرم افزار، برنامه ریزی حمل و نقل |
نوع ارائه مقاله |
ژورنال |
مجله / کنفرانس | دسترسی – IEEE Access |
دانشگاه | School of Software, Beihang University, Beijing 100083, China |
کلمات کلیدی | علوم رفتاری، برنامه های کاربردی کلان داده، مدل های پیش بینی کننده، حمل و نقل عمومی |
کلمات کلیدی انگلیسی | Behavioral sciences, big data applications, predictive models, public transportation |
شناسه دیجیتال – doi |
https://doi.org/10.1109/ACCESS.2019.2950370 |
کد محصول | E13955 |
وضعیت ترجمه مقاله | ترجمه آماده این مقاله موجود نمیباشد. میتوانید از طریق دکمه پایین سفارش دهید. |
دانلود رایگان مقاله | دانلود رایگان مقاله انگلیسی |
سفارش ترجمه این مقاله | سفارش ترجمه این مقاله |
فهرست مطالب مقاله: |
Abstract I. Introduction II. Related Work III. Proposed Methodology IV. Experiments V. Conclusion Authors Figures References |
بخشی از متن مقاله: |
Abstract
Understanding passenger behaviors is of great importance in intelligent transportation and infrastructure planning. However, the passenger trajectories are actually complex temporal data, which consist of rich spatial and temporal information. What’s more, the observed passenger trajectories may be a mixture of different types of passengers with various travelling purposes. These difficulties make the prediction of passenger behaviors a challenging work. To address these problems, this paper improves the existing passenger behavior prediction methods from the following two aspects: 1) Encoding the travelling sequence with personalized semantic sensing, and 2) constructing multi-pattern prediction models to capture multiple travelling purposes and dynamics. Along this line, this paper provides a novel passenger behavior prediction model, namely, Semantic and multi-Pattern Long Short-Term Memory (SP-LSTM) model. Particularly, 1) a translation unit is designed, which is able to encode an observed travelling sequence into a structured sequence with consideration of individual travelling semantics; 2) a multi-pattern learning schematic is proposed, which first identifies the travelling patterns of passengers and then handles different patterns with different learning modules; 3) a unified learning framework is provided to integrate the semantic sensing module and multi-pattern learning module together, and present the final prediction results. To evaluate the proposed method, this paper conducts experiments on real-world passenger travelling data. Results demonstrate the superiority of SP-LSTM over both classical and the state-of-the-art methods. Introduction Public transportation plays an important role in daily life of residents, especially in metropolises such as New York, USA and Beijing, China. On the one hand, data mining and machine learning have undergone a rapid development in the recent years, machine-learning technology powers many aspects of modern society [1]; on the other hand, we have accumulated a large amount of transportation data, such as NYC Taxi Open Data [2], Uber Trip Data, Taxi Trip Records and so on. Hence, an opportunity of improving the public transportation service by data-driven solutions and strategies has been witnessed by many scholars such as [12]–[۱۶]. This paper focuses on modelling passenger behaviors and predicting passengers’ next stations. By doing this, we can help the government and enterprises optimize to dispatch the transportation resources, and also help the citizens to circumvent the crowded stations. As shown in Fig. 1, If travelling behaviors of passengers can be predicted precisely, a better traffic route planning can be made for workers with regular moving patterns, such as P1 and P3, and recommended routes or tourism products can be provided for travelers shown as P2. |