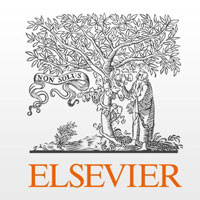
مشخصات مقاله | |
عنوان مقاله | Advance purchase behaviors of air tickets |
ترجمه عنوان مقاله | رفتارهای پیش خرید بلیط هواپیما |
فرمت مقاله | |
نوع مقاله | ISI |
نوع نگارش مقاله | مقاله پژوهشی (Research article) |
سال انتشار | |
تعداد صفحات مقاله | ۸ صفحه |
رشته های مرتبط | علوم فنون هوایی |
مجله | مجله مدیریت حمل و نقل هوایی – Journal of Air Transport Management |
دانشگاه | بخش حمل و نقل و لجستیک مدیریت، دانشگاه ملی چیائو تونگ، تایوان |
کلمات کلیدی | رفتارهای پیش خرید ، مدل لوجیت چند جمله ای، داده های تراکنشی، مدیریت درآمد |
کد محصول | E4075 |
نشریه | نشریه الزویر |
لینک مقاله در سایت مرجع | لینک این مقاله در سایت الزویر (ساینس دایرکت) Sciencedirect – Elsevier |
وضعیت ترجمه مقاله | ترجمه آماده این مقاله موجود نمیباشد. میتوانید از طریق دکمه پایین سفارش دهید. |
دانلود رایگان مقاله | دانلود رایگان مقاله انگلیسی |
سفارش ترجمه این مقاله | سفارش ترجمه این مقاله |
بخشی از متن مقاله: |
۱٫ Introduction
The principle of revenue management (RM) in the airline industry is to maximize their farebox revenue through pricing and allocating available seats under uncertain demand and perishable supply. In practice, RM implementation is usually associated with setting booking limits through different fare products. The booking limits restrain the maximum number of seats available for sale to a given booking class, whereas a fare product is a combination of a price and fare restrictions. Through setting the booking limits for each designed fare products, airlines are able to derive the optimal selling strategy based on remaining capacity, market conditions and anticipated demand. Generally, RM demand model has been proposed based on a hypothesized inverse demand function using traditional statistics techniques, such as time series, averaging methods, or simple probability distributions (McGill and van Ryzin, 1999). Those demand models mostly assume passenger demand to be independent among fare products that created based on different restrictions for passenger segmentation. However, with increasing market competition from low cost carriers (LCCs) and the growth of online ticket sales, passengers nowadays may perceive fare classes as nothing more than different prices for a seat and purchase based on price rather than fare product. That results in the RM demand forecast model assumptions, such as independence across fare products, may no longer be valid (Barnhart and Smith, 2012). Additionally, airlines employ strikingly different pricing strategies under intense market competition, differentiated demand patterns, and achieving effective customer segmentation (Bilotkach et al., 2010). For example, by setting advance purchase discount, airlines are able to induce price-sensitive passengers to purchase earlier whereas the less price-sensitive but time-sensitive passengers purchase later and further shift demand (Gallego et al., 2008; Dana, 1999, 1998; Gale and Holmes, 1993). Moreover, airlines also adjust prices dynamically based on learning demand (Escobari, 2012; Deneckere and Peck, 2012). Passengers can decide to make advance purchase at the going price or to delay their purchase decision. Those price strategies may decrease the product value that passengers are forced to make trade-offs between price, product attributes and advance purchase deadlines, and therefore, change their purchasing behaviors (Hotle et al., 2015; Escobari, 2014). Without knowing the real purchasing behaviors of air passengers, the hypothesized demand function may lead to an erroneous estimated result. In order to trace individuals’ advance purchase decisions, recent researches have introduced discrete choice models to RM for its ability to accommodate passenger preferences in RM strategies thatcan better explain how individuals making trade-offs (Garrow, 2009; Talluri and van Ryzin, 2004a, 2004b). The decision of passengers can be modeled based on either stated preferences survey data (Proussaloglou and Koppelman, 1999; Wen and Lai, 2010) or revealed preferences data. Despite that demand models based on discrete choice models may be more appropriate in RM applications, for the revealed preferences settings, there is limited empirical research due to data acquisition problems. Both chosen and non-chosen alternatives are needed for revealed preference model implementations. Although the support of computer systems lowers down data collection costs, most of firms can still only record the results of passengers of successful purchase and information about non-chosen alternatives had been difficult to obtain, which made inferring the true demand with available data remains a quite expensive and challenge issue. Previous researches estimated logit models of demand to analyze advance purchase behaviors based on revealed preferences data in airline industry (Escobari and Mellado, 2014; Vulcano et al., 2010; Carrier, 2008), hotel (Newman et al., 2014) and railway industry (Hetrakul and Cirillo, 2013, 2014, 2015). To our best knowledge, Escobari and Mellado (2014) is the first study that using seat inventory changes and posted prices to estimate the flight itinerary choice from revealed preference approach, where both chosen and nonchosen information for all alternative flights of different airlines are available. |