مقاله انگلیسی رایگان در مورد تجزیه و تحلیل داده های بزرگ و عملکرد شرکت – الزویر ۲۰۱۹
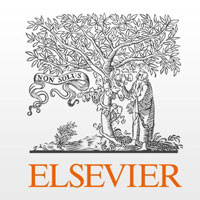
مشخصات مقاله | |
ترجمه عنوان مقاله | تجزیه و تحلیل داده های بزرگ و عملکرد شرکت: یافته هایی از رویکرد روش مخلوط |
عنوان انگلیسی مقاله | Big data analytics and firm performance: Findings from a mixed-method approach |
انتشار | مقاله سال ۲۰۱۹ |
تعداد صفحات مقاله انگلیسی | ۱۶ صفحه |
هزینه | دانلود مقاله انگلیسی رایگان میباشد. |
پایگاه داده | نشریه الزویر |
نوع نگارش مقاله |
مقاله پژوهشی (Research Article) |
مقاله بیس | این مقاله بیس میباشد |
نمایه (index) | Scopus – Master Journals List – JCR |
نوع مقاله | ISI |
فرمت مقاله انگلیسی | |
ایمپکت فاکتور(IF) |
۵٫۳۵۲ در سال ۲۰۱۸ |
شاخص H_index | ۱۵۸ در سال ۲۰۱۹ |
شاخص SJR | ۱٫۶۸۴ در سال ۲۰۱۸ |
شناسه ISSN | ۰۱۴۸-۲۹۶۳ |
شاخص Quartile (چارک) | Q1 در سال ۲۰۱۸ |
مدل مفهومی | دارد |
پرسشنامه | ندارد |
متغیر | دارد |
رفرنس | دارد |
رشته های مرتبط | مدیریت |
گرایش های مرتبط | مدیریت کسب و کار |
نوع ارائه مقاله |
ژورنال |
مجله / کنفرانس | مجله تحقیقات کسب و کار-Journal of Business Research |
دانشگاه | Department of Computer Science, Norwegian University of Science and Technology, Sem Sælandsvei 9, 7491 Trondheim, Norway |
کلمات کلیدی | تجزیه و تحلیل داده های بزرگ، نظریه پیچیدگی، تحلیل های مقایسه ای کیفی مجموعه فازی (fsQCA)، ارزش کسب و کار، روش مخلوط، عدم قطعیت محیطی |
کلمات کلیدی انگلیسی | Big data analytics، Complexity theory، fsQCA، Business value، Mixed-method، Environmental uncertainty |
شناسه دیجیتال – doi |
https://doi.org/10.1016/j.jbusres.2019.01.044 |
کد محصول | E12192 |
وضعیت ترجمه مقاله | ترجمه آماده این مقاله موجود نمیباشد. میتوانید از طریق دکمه پایین سفارش دهید. |
دانلود رایگان مقاله | دانلود رایگان مقاله انگلیسی |
سفارش ترجمه این مقاله | سفارش ترجمه این مقاله |
فهرست مطالب مقاله: |
Abstract ۱٫ Introduction ۲٫ Background and research framework ۳٫ Method ۴٫ Findings ۵٫ Discussion Acknowledgements Appendix A. Survey instrument Appendix B. Heterotrait-monotrait ratio (HMTM) Appendix C. Fuzzy set calibration Appendix D. Interview guidelines References |
بخشی از متن مقاله: |
Abstract
Big data analytics has been widely regarded as a breakthrough technological development in academic and business communities. Despite the growing number of firms that are launching big data initiatives, there is still limited understanding on how firms translate the potential of such technologies into business value. The literature argues that to leverage big data analytics and realize performance gains, firms must develop strong big data analytics capabilities. Nevertheless, most studies operate under the assumption that there is limited heterogeneity in the way firms build their big data analytics capabilities and that related resources are of similar importance regardless of context. This paper draws on complexity theory and investigates the configurations of resources and contextual factors that lead to performance gains from big data analytics investments. Our empirical investigation followed a mixed methods approach using survey data from 175 chief information officers and IT managers working in Greek firms, and three case studies to show that depending on the context, big data analytics resources differ in significance when considering performance gains. Applying a fuzzy-set qualitative comparative analysis (fsQCA) method on the quantitative data, we show that there are four different patterns of elements surrounding big data analytics that lead to high performance. Outcomes of the three case studies highlight the inter-relationships between these elements and outline challenges that organizations face when orchestrating big data analytics resources. Introduction We are living in the “Age of Data”, with new data being produced from all industries and public bodies at an unprecedented, and constantly growing rate (McAfee, Brynjolfsson, & Davenport, 2012). As a result, there has been a great hype which has led organizations to make substantial investments in their quest to explore how they can use their data to create value (Constantiou & Kallinikos, 2015). The main premise big data analytics builds on is that by analyzing large volumes of unstructured data from multiple sources, actionable insights can be generated that can help firms transform their business and gain an edge over their competition (Chen, Chiang, & Storey, 2012). Being able to obtain such data-generated insight are particularly relevant, especially for organizations that operate in dynamic and high-paced business environments, where making informed decisions is critical (Wamba et al., 2017). |