مقاله انگلیسی رایگان در مورد شبکه های اجتماعی تلفیقی و تحلیل پوششی داده ها برای رتبه بندی – الزویر ۲۰۱۷
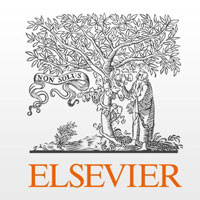
مشخصات مقاله | |
انتشار | مقاله سال ۲۰۱۷ |
تعداد صفحات مقاله انگلیسی | ۲۹ صفحه |
هزینه | دانلود مقاله انگلیسی رایگان میباشد. |
منتشر شده در | نشریه الزویر |
نوع نگارش مقاله | مقاله پژوهشی (Research article) |
نوع مقاله | ISI |
عنوان انگلیسی مقاله | Combined social networks and data envelopment analysis for ranking |
ترجمه عنوان مقاله | شبکه های اجتماعی تلفیقی و تحلیل پوششی داده ها برای رتبه بندی |
فرمت مقاله انگلیسی | |
رشته های مرتبط | مهندسی فناوری اطلاعات |
گرایش های مرتبط | اینترنت و شبکه های گسترده |
مجله | مجله اروپایی تحقیقات عملیاتی – European Journal of Operational Research |
دانشگاه | Departament of Computer’s Sciences – Rey Juan Carlos University – Spain |
کلمات کلیدی | تحلیل پوششی داده ها، شبکه های اجتماعی، رتبه بندی، معیار سنجش، PageRank |
کلمات کلیدی انگلیسی | Data envelopment analysis, Social networks, Rankings, Benchmarking, PageRank |
شناسه دیجیتال – doi |
https://doi.org/10.1016/j.ejor.2017.10.025 |
کد محصول | E8604 |
وضعیت ترجمه مقاله | ترجمه آماده این مقاله موجود نمیباشد. میتوانید از طریق دکمه پایین سفارش دهید. |
دانلود رایگان مقاله | دانلود رایگان مقاله انگلیسی |
سفارش ترجمه این مقاله | سفارش ترجمه این مقاله |
بخشی از متن مقاله: |
۱٫ Introduction
Data Envelopment Analysis (also known by its initials DEA) is a common method, used by in a large number of studies on benchmarking, for assessing the efficiency of organizations, introduced by Charnes, Cooper, and Rhodes (1978), DEA is based on a methodology that measures the relative efficiencies of a given group of organizations, classifying them as efficient and inefficient. The criterion used for this classification is determined by the location of each organization with respect to the efficient production frontier. This frontier is formed by the units that represent best management practices, in regards to outputs and the resources used in their production. Units identified as inefficient can be compared to one or more units located on the frontier, which can be considered as benchmarks for guiding improvement efforts (Bergendahl, 1998). However, an important problem in the application of DEA for ranking is that usually all efficient units are given the same efficiency score of one (Cook & Seiford, 2009), there being, to date, no agreement over the best methodology for ordering or classifying efficient units by importance. Some, standard measures used in DEA for ranking efficient and inefficient units are worth mentioning: counting the number of times a particular efficient company acts as a reference DMU (Zhu, 2000), calculating a super- efficiency measure (Andersen & Petersen, 1993; Bardhan et al 1996; Tone, 2002; Chen, 2005), calculating a peer index (Torgersen, Forsund, & Kittelsen, 1996, Zhu, 2000), weight restrictions (Dyson & Thanassoulis, 1988; Charnes et al., 1990; Thompson et al. 1986; Wong & Beasley, 1990), value efficiency analysis (Halme et al., 2000), cross-evaluation (Doyle & Green, 1994), multiple objective linear programming (Li & Reeves, 1999), multivariate statistical techniques (Friedman & Sinuany-Stern, 1997, Sinuany-Stern, Mehrez & Barboy, 1994), and conditional directional distance (Daraio, Bonaccorsi & Simar, 2015). For a good summary of such ranking techniques see: AnguloMeza and Lins (2002); Adler, Friedman, and Sinuany-Stern (2002); Lu and Lo (2009); and Lotfi et al. (2013). Since DEA is applied to DMUs groups with homogenous characteristics, it has been considered convenient to use Social Network Analysis (SNA) to identify the importance of each DMU within the network. Nevertheless, the use of SNA analysis in DEA ranking problems has been limited by the way in which the DEA network is modeled or the centrality measure considered. Liu, Lu, Yang, and Chuang (2009), use the eigenvector centrality measure proposed by Bonacich and Lloyd (2001) on a social network, that is built by adding the lambda values associated with the reference DMUs, obtained by applying DEA to all of the possible input and output combinations. Liu and Lu (2010) modified this approach to correct the convergence of the proposed algorithm and Leen and Chun (2015) use PageRank (Brin & Page, 1998), the algorithm behind Google’s search engine, for determining the influence and rank of efficient DMUs. |