مقاله انگلیسی رایگان در مورد یادگیری عمیق برای برچسب گذاری و طبقه بندی موسیقی مبتنی بر صوت – ۲۰۱۹ IEEE
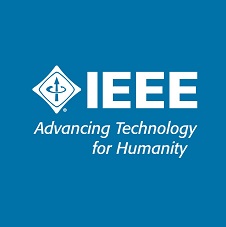
مشخصات مقاله | |
ترجمه عنوان مقاله | یادگیری عمیق برای برچسب گذاری و طبقه بندی موسیقی مبتنی بر صوت: آموزش کامپیوتر برای تشخیص راک از باخ |
عنوان انگلیسی مقاله | Deep Learning for Audio-Based Music Classification and Tagging: Teaching Computers to Distinguish Rock from Bach |
انتشار | مقاله سال ۲۰۱۹ |
تعداد صفحات مقاله انگلیسی | ۱۱ صفحه |
هزینه | دانلود مقاله انگلیسی رایگان میباشد. |
پایگاه داده | نشریه IEEE |
مقاله بیس | این مقاله بیس نمیباشد |
نمایه (index) | Scopus – JCR – Master Journal List |
نوع مقاله | ISI |
فرمت مقاله انگلیسی | |
ایمپکت فاکتور(IF) |
۷٫۰۹۷ در سال ۲۰۱۸ |
شاخص H_index | ۱۵۵ در سال ۲۰۱۹ |
شاخص SJR | ۱٫۳۶۴ در سال ۲۰۱۸ |
شناسه ISSN | ۱۰۵۳-۵۸۸۸ |
شاخص Quartile (چارک) | Q1 در سال ۲۰۱۸ |
رشته های مرتبط | مهندسی کامپیوتر |
گرایش های مرتبط | مهندسی نرم افزار، هوش مصنوعی |
نوع ارائه مقاله |
ژورنال |
مجله | مجله پردازش سیگنال – Signal Processing Magazine |
دانشگاه | Culture Technology, Korea Advanced Institute of Science and Technology, Daejeon, South Korea |
شناسه دیجیتال – doi |
https://doi.org/10.1109/MSP.2018.2874383 |
کد محصول | E11422 |
وضعیت ترجمه مقاله | ترجمه آماده این مقاله موجود نمیباشد. میتوانید از طریق دکمه پایین سفارش دهید. |
دانلود رایگان مقاله | دانلود رایگان مقاله انگلیسی |
سفارش ترجمه این مقاله | سفارش ترجمه این مقاله |
فهرست مطالب مقاله: |
۱- From feature engineering to end-to-end learning
۲- Deep-learning models ۳- Data sets and tasks ۴- Practical guide ۵- Applications ۶- Limitations and future challenges References |
بخشی از متن مقاله: |
From feature engineering to end-to-end learning Humans classify or annotate music based on diverse characteristics extracted from the audio signals. For example, a heavily distorted electric guitar sound with growling vocals is a good indication of metal music. Swing rhythms, syncopation, and chromatic comping by polyphonic instruments (e.g., piano or guitars) are obvious cues that the music is jazz. Translating these acoustic and musical features into numerical representations that computers can interpret is the essence of music classification and tagging. This usually involves a series of computation steps that convert audio content into a time–frequency representation, extract discriminative features, summarize them over time, and repeat the feature extraction and summarization until the proper category for the music can be determined. The way of improving each feature extraction step to achieve the best performance has evolved with advances in learning algorithms from hand engineering based on domain knowledge to end-to-end learning. Humphrey et al. [9] explained the transition in a unified deep architecture model where multiple blocks of affine transformation, nonlinear function, and optional pooling operation are pipelined. Figure 1 illustrates four different feature representation approaches in their framework. In reviewing the evolution of such approaches, we first separate them into two classes: feature engineering and feature learning. |