مقاله انگلیسی رایگان در مورد شبکه تشخیص آتش سوزی سبک وزن غیر موقت – IEEE 2019
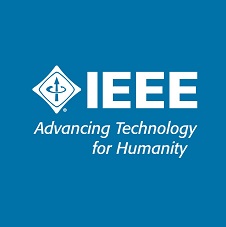
مشخصات مقاله | |
ترجمه عنوان مقاله | شبکه تشخیص آتش سوزی سبک وزن غیر موقت برای سیستم های هوشمند نظارتی |
عنوان انگلیسی مقاله | Non-Temporal Lightweight Fire Detection Network for Intelligent Surveillance Systems |
انتشار | مقاله سال ۲۰۱۹ |
تعداد صفحات مقاله انگلیسی | ۱۰ صفحه |
هزینه | دانلود مقاله انگلیسی رایگان میباشد. |
پایگاه داده | نشریه IEEE |
نوع نگارش مقاله |
مقاله پژوهشی (Research Article) |
مقاله بیس | این مقاله بیس نمیباشد |
نمایه (index) | Scopus – Master Journals List – JCR |
نوع مقاله | ISI |
فرمت مقاله انگلیسی | |
ایمپکت فاکتور(IF) |
۴٫۶۴۱ در سال ۲۰۱۸ |
شاخص H_index | ۵۶ در سال ۲۰۱۹ |
شاخص SJR | ۰٫۶۰۹ در سال ۲۰۱۸ |
شناسه ISSN | ۲۱۶۹-۳۵۳۶ |
شاخص Quartile (چارک) | Q2 در سال ۲۰۱۸ |
مدل مفهومی | ندارد |
پرسشنامه | ندارد |
متغیر | ندارد |
رفرنس | دارد |
رشته های مرتبط | مهندسی کامپیوتر، مهندسی فناوری اطلاعات |
گرایش های مرتبط | هوش مصنوعی، شبکه های کامپیوتری |
نوع ارائه مقاله |
ژورنال |
مجله / کنفرانس | دسترسی – IEEE Access |
دانشگاه | Department of Electronic Engineering, Inha University, Incheon 22212, South Korea |
کلمات کلیدی | تشخیص آتش، یادگیری عمیق، شبکه های عصبی پیچشی، طبقه بندی تصویر |
کلمات کلیدی انگلیسی | Fire detection, deep learning, convolutional neural networks, image classification |
شناسه دیجیتال – doi |
https://doi.org/10.1109/ACCESS.2019.2953558 |
کد محصول | E14048 |
وضعیت ترجمه مقاله | ترجمه آماده این مقاله موجود نمیباشد. میتوانید از طریق دکمه پایین سفارش دهید. |
دانلود رایگان مقاله | دانلود رایگان مقاله انگلیسی |
سفارش ترجمه این مقاله | سفارش ترجمه این مقاله |
فهرست مطالب مقاله: |
Abstract I. Introduction II. Proposed Algorithm III. Experiments IV. Robustness Analysis V. Conclusion Authors Figures References |
بخشی از متن مقاله: |
Abstract
Convolutional neural networks (CNNs) have been recently applied to tackle a variety of computer vision problems. However, because of its high computational cost, careful considerations are required to design cost-effective CNNs. In this paper, we propose a CNN inspired by MobileNet for fire detection in surveillance systems. In the proposed network, color features emphasized by the channel multiplier are extracted through depthwise separable convolution, and squeeze and excitation modules further increase the representation of the channel-wise convolution. Custom Swish is used as an activation function to limit exceedingly high weights from the effects of the channel multiplier. Our proposed network achieves 95.44% accuracy for fire detection, which is higher than those achieved other existing networks. Furthermore, the number of parameters used is 38.50% fewer than that of MobileNetV2, the smallest among other networks. We believe that using the proposed CNN, CNN-based surveillance systems could be implemented in lightweight devices without using expensive dedicated processors. Introduction Fires can occur anywhere, at any time, and if they are not detected early, they can cause severe damages to property and people. Surveillance systems consisting of multiple CCTVs can be very useful in detecting fires because they are designed to monitor the surroundings 24 hours a day. Furthermore, they can be very useful to monitor fires in a wide range of areas, including inaccessible areas. Consequently, there has been a huge demand for intelligent video-based fire monitoring systems that can alert people to respond quickly by processing and analyzing video streams in real-time. A video-based fire detection system can inform an operator by analyzing videos from CCTVs without using heat, smoke, or flame sensors. Owing to the significant development of video analysis, video signals from CCTVs can be automatically analyzed and can provide alarms to surveillance personnel to enable quick response. Traditional vision-based fire detection methods use handcrafted features, such as color, motion, and texture. Prior studies [1]–[۴] detected fire by making full use of color features because fire is generally brighter and has higher contrast than other objects. Ko et al. [1] detected specific fire regions from their color and, then employed a model using wavelet coefficients to detect fire with a support vector machine (SVM) classifier. |