مقاله انگلیسی رایگان در مورد ساختار شبکه برنامه کاربردی برای خطوط هوایی
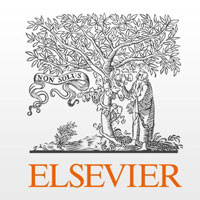
مشخصات مقاله | |
عنوان مقاله | Dynamic DEA models with network structure: An application for Iranian airlines |
ترجمه عنوان مقاله | مدل های دینامیک DEA با ساختار شبکه: یک برنامه کاربردی برای خطوط هوایی ایران |
فرمت مقاله | |
نوع مقاله | ISI |
نوع نگارش مقاله | مقاله پژوهشی (Research article) |
سال انتشار | |
تعداد صفحات مقاله | ۱۰ صفحه |
رشته های مرتبط | علوم فنون هوایی |
مجله | مجله مدیریت حمل و نقل هوایی – Journal of Air Transport Management |
دانشگاه | دانشکده مهندسی صنایع، دانشگاه صنعتی ارومیه، ایران |
کلمات کلیدی | بهره وری، شبکه پویا DEA ، خطوط هوایی، تجزیه و تحلیل رابطه |
کد محصول | E4074 |
نشریه | نشریه الزویر |
لینک مقاله در سایت مرجع | لینک این مقاله در سایت الزویر (ساینس دایرکت) Sciencedirect – Elsevier |
وضعیت ترجمه مقاله | ترجمه آماده این مقاله موجود نمیباشد. میتوانید از طریق دکمه پایین سفارش دهید. |
دانلود رایگان مقاله | دانلود رایگان مقاله انگلیسی |
سفارش ترجمه این مقاله | سفارش ترجمه این مقاله |
بخشی از متن مقاله: |
۱٫ Introduction
Charnes et al. (1978) introduced data envelopment analysis (DEA) to measure the efficiency of DMUs with multiple inputs and multiple outputs. DEA considers the system as a black box, which ignores internal relations of processes. In the real world, DMU’s structure may contain several connected processes. One of the most important approaches for evaluating the system with several processes is network DEA (NDEA) model, which takes into account the component processes and their internal relations via intermediate products in measuring efficiency. Fare and Grosskopf (2000) proposed network DEA models to evaluate the performance of organization and its component processes. Their model considered the processes as independent ones in the network, so no mathematical relationship does exist between the system and process effi- ciencies. To overcome the problem, Kao (2009) proposed relational network DEA model. This model takes into account the relationship of the processes, to measure the system and process efficiencies at the same time and obtained a relationship between system and process efficiencies. Independent models which calculate the process efficiencies independently, allow a factor (input/output/link) to have different multipliers in different places, but the relational model requires the same factor to have the same multiplier associated with it, regardless of the place it corresponds to. The network systems are classified in various structures such as two-stage, series, parallel and mixed (Kao and Hwang, 2010). Models used to measure the efficiency of network systems are classified in several types, such as independent models, distance measure model, slacks-based measure model, ratio-form model, game theoretic model and value-based model (Kao, 2014). Tone and Tsutsui (2009) measured the efficiency of network systems by a slack-based measure (SBM) DEA model which can decompose the system effi- ciency into processes efficiency. For evaluating the processes of the system, Fare and Grosskopf (1996) considered the production system consisting of independent processes and calculated the effi- ciency of processes, separately. In the real world, companies have long-term planning, so dynamic models are needed to consider inter-relationships between single periods, which are any kind of flows, to assess the performance of DMUs over time. The capital inputs that generate outputs in the future are suitable for explaining dynamic aspects of systems to measure the efficiency,appropriately. In the inter-temporal case, the capital inputs change along the optimal path of capacity expansion yielding a long-run production frontier (Sengupta, 1994). Sengupta (1994) developed an adjustment cost approach which modifies the standard model of production frontier to analyze risk aversion behavior of the DMUs on the dynamic production frontier, but the proposed system had one output. Fare et al. (1996) presented a discussion of dynamic structures with multiple outputs and introduced dynamic aspect of production on DEA model. They developed a sequence of network models that became the base for further studies on dynamic DEA. Most of the studies in dynamic systems calculate overall effi- ciency and period-specific efficiencies, separately. Jaenicke (2000) used dynamic DEA (DDEA) analysis to model the dynamic production technology associated with relational crop production. In his study, soil capital was considered as intermediate output of model. The model measures efficiency of rotation made up from three kinds of crops. Tone and Tsutsui (2010) developed dynamic DEA model in slack-based measure framework that deals with inputs and outputs individually (such non-radial models). Kao (2013) presented a relational model for dynamic systems to calculate the radial measures of the overall and period efficiencies of multiperiod production system where consecutive periods are connected by flows. Radial approaches assume proportional changes in inputs or outputs. Chen (2009) proposed a new approach named “j-efficiency measure” to incorporate the dynamic effect within the production network, systematically. This approach estimates the efficiencies of sub-DMUs (SDMUs), then the efficiency of the entire DMU. j is defined as input-oriented efficiency indices of SDMU that represents the minimal aggregate input requirement with respect to the aggregate final output in the periods (Chen, 2009). Chen (2012) proposed a dynamic multi-activity network DEA (DMNDEA) model to determine the performance of farrow-to- finish swine production in Taiwan. The production was consisting of two processes; the breed-to-farrow and wean-to-finish. Chen (2012) applied a distance function to construct DMNDEA model and calculated the overall and process efficiencies of pig farms. Distance function considers the distance of DMU’s current condition from the ideal condition (frontier) for calculating the efficiency. Tone and Tsutsui (2014) proposed dynamic DEA models for network structures within the slack-based measure (SBM) to evaluate the performance of a company and applied the model to a dataset of US electric companies over multiple years. The SBM approach uses slack variables for calculating the efficiency scores and no relationship is defined between system and processes effi- ciency. In this paper, a radial dynamic DEA model with network structure is presented to observe dynamic changes of both subsystem and period efficiency. It is notable that the relational models obtain the relationship between system and processes inefficiency to configure the source of inefficiency in system, whereas the SBMDNDEA model presented by Tone and Tsutsui (2014) is not capable to. The reason for developing DNDEA model is that in real problems the performance of DMU’s internal divisions relies on several periods. For example in a supply chain consisting of supplier, producer and distributor, it’s possible that at the end of the year production division has excess inventory in its warehouse. Thus, extra stock will be sent to distributor at the next year. The main contribution of this paper is combination of the relational DDEA model introduced by Kao (2013) and the relational NDEA model presented by Kao and Hwang (2010) to present the DNDEA model. The proposed dynamic network (DNDEA) model is applied to calculate the efficiency scores of Iranian airlines and the results of dynamic (DDEA) and network (NDEA) models are compared. The rest of this paper organized as follows: Section 2 presents the NDEA model. Section 3 outlines relational dynamic DEA model graphically and its mathematical formulations. In section 4, our model is proposed and the case of Iranian airline companies is presented in section 5. Section 6 shows the results of our proposed model and the contribution of this paper is discussed in the conclusions. |