مقاله انگلیسی رایگان در مورد تقسیم حالت IMF در EMD برای تحلیل jitter (الزویر)
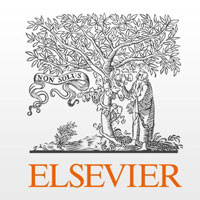
مشخصات مقاله | |
انتشار | مقاله سال ۲۰۱۶ |
تعداد صفحات مقاله انگلیسی | ۱۳ صفحه |
هزینه | دانلود مقاله انگلیسی رایگان میباشد. |
منتشر شده در | نشریه الزویر |
نوع مقاله | ISI |
عنوان انگلیسی مقاله | IMF mode demixing in EMD for jitter analysis |
ترجمه عنوان مقاله | تقسیم حالت IMF در EMD برای تحلیل jitter |
فرمت مقاله انگلیسی | |
رشته های مرتبط | اقتصاد |
گرایش های مرتبط | اقتصاد پولی |
مجله | مجله علوم محاسباتی – Journal of Computational Science |
دانشگاه | Department of Software Engineering – Kaunas University of Technology – Kaunas – Lithuania |
کلمات کلیدی | پردازش سیگنال دیجیتال انهدام سیگنال، تجزیه حالت تجربی، حالت مخلوط کردن، تحلیل جرثقیل |
کد محصول | E5267 |
وضعیت ترجمه مقاله | ترجمه آماده این مقاله موجود نمیباشد. میتوانید از طریق دکمه پایین سفارش دهید. |
دانلود رایگان مقاله | دانلود رایگان مقاله انگلیسی |
سفارش ترجمه این مقاله | سفارش ترجمه این مقاله |
بخشی از متن مقاله: |
I. INTRODUCTION
Signal decomposition (or source separation) is important in many domains of application (such as telecommunications, speech recognition, biomedical signal analysis, computer vision, seismic signal processing, time series forecasting), where several signals (including noise) have been mixed together into a combined signal and the aim is to recover the original constituents from the mixture signal. Several approaches can be used to perform signal decomposition such as Wavelet Transform [1], Independent Component Analysis (ICA) [2], Prony decomposition [3], Vold-Kalman filter (VKF) [4], blind decorrelation [5], multichannel decorrelation [6], Time Delayed Correlations [7], Denoising Source Separation [8], second generation wavelets [9], generalized eigenvalue decomposition [10], Quadratic Component Analysis [11], Fractal decomposition [12], Singular Spectrum Decomposition (SSD) [13], Biorthogonal Wavelet Decomposition (BWD) [14], Fourier Decomposition (FD) [15], Structural Sparse Decomposition [16], Regular Decomposition [17], Principle Phase Decomposition [18], Singular Value Decomposition (SVD) [19], Intrinsic Mode Decomposition [20], Pulse-Amplitude Modulated (PAM) decomposition [21], Variational Mode Decomposition [22], Transient Decomposition [23], Scattering Decomposition [24], Autoregressive Decomposition [25] and to separate informative and noise signal components. However, the existing methods for signal decomposition have a number of limitations and shortcomings. For example, while Fourier Transform is effective when applied to stationary signals, it makes little physical sense when applied to non-stationary and noisy data |