مقاله انگلیسی رایگان در مورد ۱۰ روش برتر داده کاوی در کاربرد های تجاری – امرالد ۲۰۱۷
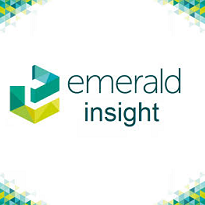
مشخصات مقاله | |
انتشار | مقاله سال ۲۰۱۷ |
تعداد صفحات مقاله انگلیسی | ۲۱ صفحه |
هزینه | دانلود مقاله انگلیسی رایگان میباشد. |
منتشر شده در | نشریه امرالد |
نوع مقاله | ISI |
عنوان انگلیسی مقاله | Top 10 Data Mining Techniques in Business Applications: A Brief Survey |
ترجمه عنوان مقاله | ۱۰ روش برتر داده کاوی در کاربرد های تجاری: یک تحقیق خلاصه |
فرمت مقاله انگلیسی | |
رشته های مرتبط | مهندسی صنایع |
گرایش های مرتبط | داده کاوی |
مجله | Kybernetes |
دانشگاه | Asia University – Taichung – Taiwan |
کلمات کلیدی | داده کاوی؛ برنامه های کاربردی تجاری؛ فراگیری ماشین؛ بررسی |
کلمات کلیدی انگلیسی | data mining; business applications; machine learning; survey |
کد محصول | E7079 |
وضعیت ترجمه مقاله | ترجمه آماده این مقاله موجود نمیباشد. میتوانید از طریق دکمه پایین سفارش دهید. |
دانلود رایگان مقاله | دانلود رایگان مقاله انگلیسی |
سفارش ترجمه این مقاله | سفارش ترجمه این مقاله |
بخشی از متن مقاله: |
۱٫ Introduction
Data are key assets to any business enterprise, which means that techniques for data mining and knowledge discovery in databases (KDD) play an important role in many business applications and assist with the making of effective decisions. The aim is to identify useful and/or interesting knowledge from a given dataset, which can then be treated as a decision support system. In general, the process is comprised of four steps: data collection, data cleaning and preprocessing, data analysis, and interpretation and evaluation (Han and Kamber, 2001; Ye, 2003). Of these steps, data analysis is essential to the performance of data mining tasks, based on employing relevant statistical and/or machine learning techniques. In early surveys of Wong et al. (1997) and Vellido et al. (1999), artificial neural networks were recognized as one of the most popularly used data mining algorithms for a diverse range of business applications in the 80s and 90s. Bose and Mahapatra (2001) reviewed a number of machine learning techniques in the context of mining business data. They found rule induction (i.e., decision trees) to be the most commonly used technique, followed by artificial neural networks and case-based reasoning. In addition, most applications were found in areas related to finance, where prediction of future eventualities is the dominant task category for business data mining. Recently, there have been several studies surveying the data mining techniques used in specific business applications. These surveys also show that the artificial neural network is one of the widely employed techniques for various business problems, such as fraud detection (Ngai et al., 2011), bankruptcy prediction and credit scoring (Lin et al., 2012), recommender systems (Bobadilla et al., 2013), and software development effort estimation (Wen et al., ۲۰۱۲). Wu et al. (2008) has identified the top (or frequently used) 10 data mining algorithms, which covered classification (including C4.5, SVM, AdaBoost, k-NN, naïve Bayes, and CART), clustering (including k-means and EM), statistical learning (i.e., EM and Naïve Bayes), association analysis (i.e., Apriori), and link mining (i.e., PageRank). In particular, they focus on describing these algorithms, discussing the impact of these algorithms, and reviewing current and future research on these algorithms. However, the status of current data mining algorithms for business applications is not analyzed. With the exception of the work of Bose and Mahapatra (2001) the purpose of these surveys has not been to answer the question of what are the top data mining techniques for popular business applications. More specifically, they only describe what kinds of techniques were used for which business domain problems. In addition, their survey of data mining techniques and business application areas is not new, where the works were published before 1999. |