مقاله انگلیسی رایگان در مورد قیاس های واژه بین زبانی – الزویر ۲۰۱۹
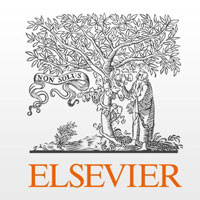
مشخصات مقاله | |
ترجمه عنوان مقاله | قیاس های واژه بین زبانی با استفاده از تحولات خطی بین فضاهای معنایی |
عنوان انگلیسی مقاله | Cross-lingual word analogies using linear transformations between semantic spaces |
انتشار | مقاله سال ۲۰۱۹ |
تعداد صفحات مقاله انگلیسی | ۹ صفحه |
هزینه | دانلود مقاله انگلیسی رایگان میباشد. |
پایگاه داده | نشریه الزویر |
نوع نگارش مقاله |
مقاله پژوهشی (Research Article) |
مقاله بیس | این مقاله بیس نمیباشد |
نمایه (index) | Scopus – Master Journals List – JCR |
نوع مقاله | ISI |
فرمت مقاله انگلیسی | |
ایمپکت فاکتور(IF) |
۵٫۸۹۱ در سال ۲۰۱۸ |
شاخص H_index | ۱۶۲ در سال ۲۰۱۹ |
شاخص SJR | ۱٫۱۹۰ در سال ۲۰۱۸ |
شناسه ISSN | ۰۹۵۷-۴۱۷۴ |
شاخص Quartile (چارک) | Q1 در سال ۲۰۱۸ |
مدل مفهومی | ندارد |
پرسشنامه | ندارد |
متغیر | ندارد |
رفرنس | دارد |
رشته های مرتبط | مهندسی کامپیوتر |
گرایش های مرتبط | معماری سیستم های کامپیوتری |
نوع ارائه مقاله |
ژورنال |
مجله / کنفرانس | سیستم های خبره با کابردهای مربوطه – Expert Systems with Applications |
دانشگاه | NTIS – New Technologies for the Information Society, Faculty of Applied Sciences, University of West Bohemia, Pilsen, Czech Republic |
کلمات کلیدی | قیاس های واژه، فضاهای معنایی، تحولات خطی، تعبیه واژه، فضاهای معنایی بین زبانی |
کلمات کلیدی انگلیسی | Word analogies، Semantic spaces، Linear transformation، Word embeddings، Cross-lingual semantic spaces |
شناسه دیجیتال – doi |
https://doi.org/10.1016/j.eswa.2019.06.021 |
کد محصول | E13571 |
وضعیت ترجمه مقاله | ترجمه آماده این مقاله موجود نمیباشد. میتوانید از طریق دکمه پایین سفارش دهید. |
دانلود رایگان مقاله | دانلود رایگان مقاله انگلیسی |
سفارش ترجمه این مقاله | سفارش ترجمه این مقاله |
فهرست مطالب مقاله: |
Abstract ۱٫ Introduction ۲٫ Linear transformations between semantic spaces ۳٫ Cross-lingual word analogies ۴٫ Experiments ۵٫ Summary Disclosure of conflict of interest CRediT authorship contribution statement Acknowledgments References |
بخشی از متن مقاله: |
Abstract
The ability to represent the meaning of words is one of the core parts of natural language understanding (NLU), with applications ranging across machine translation, summarization, question answering, information retrieval, etc. The need for reasoning in multilingual contexts and transferring knowledge in crosslingual systems has given rise to cross-lingual semantic spaces, which learn representations of words across different languages. With growing attention to cross-lingual representations, it has became crucial to investigate proper evaluation schemes. The word-analogy-based evaluation has been one of the most common tools to evaluate linguistic relationships (such as male-female relationships or verb tenses) encoded in monolingual meaning representations. In this paper, we go beyond monolingual representations and generalize the word analogy task across languages to provide a new intrinsic evaluation tool for cross-lingual semantic spaces. Our approach allows examining cross-lingual projections and their impact on different aspects of meaning. It helps to discover potential weaknesses or advantages of cross-lingual methods before they are incorporated into different intelligent systems. We experiment with six languages within different language families, including English, German, Spanish, Italian, Czech, and Croatian. State-of-the-art monolingual semantic spaces are transformed into a shared space using dictionaries of word translations. We compare several linear transformations and rank them for experiments with monolingual (no transformation), bilingual (one semantic space is transformed to another), and multilingual (all semantic spaces are transformed onto English space) versions of semantic spaces. We show that tested linear transformations preserve relationships between words (word analogies) and lead to impressive results. We achieve average accuracy of 51.1%, 43.1%, and 38.2% for monolingual, bilingual, and multilingual semantic spaces, respectively. Introduction Word distributional-meaning representations have been the key in recent success in various natural language processing (NLP) tasks. The fundamental assumption (Distributional Hypothesis) is that two words are expected to be semantically similar if they occur in similar contexts (they are similarly distributed across the text). This hypothesis was formulated by Harris (1954) several decades ago. Today it is the basis of state-of-the-art distributional semantic models (Bojanowski, Grave, Joulin, & Mikolov, 2017; Mikolov, Chen, Corrado, & Dean, 2013a; Pennington, Socher, & Manning, 2014; Salle, Villavicencio, & Idiart, 2016). These models learn similar semantic vectors for similar words during training. In addition, the vectors capture rich linguistic relationships such as male-female relationships or verb tenses. Such vectors can significantly improve generalization when used as features in various systems, e.g., named entity recognition (Konkol, Brychcín, & Konopík, 2015), sentiment analysis (Hercig, Brychcín, Svoboda, Konkol, & Steinberger, 2016), dialogue act recognition (Brychcín & Král, 2017), etc. The plain-text corpora are easily available in many languages, yet the manually labeled data (e.g., text annotated with named entities, syntactic dependency trees, etc.) is expensive and mostly available for mainstream languages such as English. Pan and Yang (2010) summarized the transfer learning techniques that can learn to map (to some degree) hand-crafted features from one domain to another. In general, it is difficult to design good features which generalize well across tasks and even more difficult across different languages. |