مقاله انگلیسی رایگان در مورد تحلیل ویژگی های شبکه وسیله نقلیه به کمک کلان داده و طراحی مدل های تحرک – اسپرینگر ۲۰۱۸
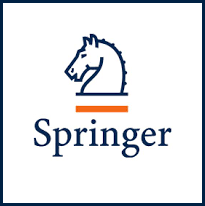
مشخصات مقاله | |
ترجمه عنوان مقاله | تجزیه و تحلیل ویژگی های شبکه وسیله نقلیه به کمک کلان داده و طراحی مدل های تحرک |
عنوان انگلیسی مقاله | Big Data Aided Vehicular Network Feature Analysis and Mobility Models Design |
انتشار | مقاله سال ۲۰۱۸ |
تعداد صفحات مقاله انگلیسی | ۹ صفحه |
هزینه | دانلود مقاله انگلیسی رایگان میباشد. |
پایگاه داده | نشریه اسپرینگر |
مقاله بیس | این مقاله بیس نمیباشد |
نمایه (index) | Scopus – Master Journal List – JCR |
نوع مقاله | ISI |
فرمت مقاله انگلیسی | |
ایمپکت فاکتور(IF) |
۲٫۸۵۰ در سال ۲۰۱۸ |
شاخص H_index | ۷۹ در سال ۲۰۱۹ |
شاخص SJR | ۰٫۴۲۶ در سال ۲۰۱۸ |
شناسه ISSN | ۱۵۷۲-۸۱۵۳ |
شاخص Quartile (چارک) | Q2 در سال ۲۰۱۸ |
رشته های مرتبط | مهندسی فناوری اطلاعات |
گرایش های مرتبط | شبکه های کامپیوتری، مدیریت سیستم های اطلاعاتی |
نوع ارائه مقاله |
ژورنال |
مجله | شبکه های موبایل و برنامه های کاربردی – Mobile Networks and Applications |
دانشگاه | Department of Electronic Engineering, Tsinghua University, China Transport Telecommunications and Information Center, Beijing, 100084, China |
کلمات کلیدی | کلان داده، شبکه وسیله نقلیه، شبکه پیچیده، مدل های تحرک |
کلمات کلیدی انگلیسی | Big data، Vehicular network، Complex network، Mobility models |
شناسه دیجیتال – doi |
https://doi.org/10.1007/s11036-017-0981-z |
کد محصول | E11262 |
وضعیت ترجمه مقاله | ترجمه آماده این مقاله موجود نمیباشد. میتوانید از طریق دکمه پایین سفارش دهید. |
دانلود رایگان مقاله | دانلود رایگان مقاله انگلیسی |
سفارش ترجمه این مقاله | سفارش ترجمه این مقاله |
فهرست مطالب مقاله: |
Abstract
۱- Introduction ۲- State-of-the-art ۳- Complex Network Feature Verification ۴- System Model ۵- Scheme Comparison ۶- Conclusion References |
بخشی از متن مقاله: |
Abstract Vehicular networks play a pivotal role in intelligent transportation system (ITS) and smart city (SC) construction, especially with the coming of 5G. Mobility models are crucial parts of vehicular network, especially for routing policy evaluation as well as traffic flow management. The big data aided vehicle mobility analysis and design attract researchers a lot with the acceleration of big data technology. Besides, complex network theory reveals the intrinsic temporal and spatial characteristics, considering the dynamic feature of vehicular network. In the following content, a big GPS dataset in Beijing, and its complex features verification are introduced. Some novel vehicle and location collaborative mobility schemes are proposed relying on the GPS dataset. We evaluate their performance in terms of complex features, such as duration distribution, interval time distribution and temporal and spatial characteristics. This paper elaborates upon mobility design and graph analysis of vehicular networks. Introduction Nowadays, large population and heavy traffic in urban areas lead to traffic congestion and automobile exhaust, reducing people’s travel experience by a large scale [1]. To address these issue, many schemes have been proposed, and vehicular networks are one of them [2]. Furthermore, vehicular networks also play a pivotal role in intelligent transportation system (ITS) and smart city (SC) construction [3], which means a lot in terms of traffic flow management [4], urban planing [5], location based recommendation service [6], etc. The arrival of 5G era provide vast potential for future development for vehicular network. In other words, there will be a greater bandwidth, higher carrier frequency, extreme base station and device densities [7, 8]. Therefore, how to design and optimize vehicular networks has been concerned by many scholars and researchers. Mobility models are of great importance to vehicular network with the consideration that vehicular network is dynamic [9]. Actually, mobility models determine its spatial and temporal characteristics of the network topology. Hence, a practical mobility model is important for assessing relevant algorithms and systems, especially for routing policy evaluation as well as traffic flow management [10]. An inappropriate mobility model may even lead to erroneous conclusions [11]. In fact, we give the way of combining the two classical mobility model construction methods. The classic mobility model is based on math, such as RWP. Pure data driven approach does not have a certain of universality. Our method aims to combine the two methods. |