مقاله انگلیسی رایگان در مورد شبکه عصبی مصنوعی برای پیش بینی تشنج با استفاده از الکتروانسفالوگرام – الزویر ۲۰۱۸
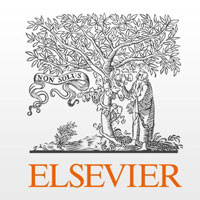
مشخصات مقاله | |
ترجمه عنوان مقاله | شبکه عصبی مصنوعی برای پیش بینی تشنج با استفاده از الکتروانسفالوگرام داخل جمجمه و پوست سر |
عنوان انگلیسی مقاله | Convolutional neural networks for seizure prediction using intracranial and scalp electroencephalogram |
انتشار | مقاله سال ۲۰۱۸ |
تعداد صفحات مقاله انگلیسی | ۲۷ صفحه |
هزینه | دانلود مقاله انگلیسی رایگان میباشد. |
پایگاه داده | نشریه الزویر |
نوع نگارش مقاله |
مقاله پژوهشی (Research Article) |
مقاله بیس | این مقاله بیس نمیباشد |
نمایه (index) | MedLine – Scopus – Master Journal List – JCR |
نوع مقاله | ISI |
فرمت مقاله انگلیسی | |
ایمپکت فاکتور(IF) |
۸٫۴۴۶ در سال ۲۰۱۷ |
شاخص H_index | ۱۲۱ در سال ۲۰۱۹ |
شاخص SJR | ۲٫۳۵۹ در سال ۲۰۱۷ |
شناسه ISSN | ۰۸۹۳-۶۰۸۰ |
شاخص Quartile (چارک) | Q1 در سال ۲۰۱۷ |
رشته های مرتبط | مهندسی کامپیوتر، فناوری اطلاعات، پزشکی |
گرایش های مرتبط | هوش مصنوعی، شبکه های کامپیوتری، مغز و اعصاب |
نوع ارائه مقاله |
ژورنال |
مجله | شبکه های عصبی – Neural Networks |
دانشگاه | School of Electrical and Information Engineering – University of Sydney – Australia |
کلمات کلیدی | پیش بینی تشخیص، شبکه عصبی کانولوشن، یادگیری ماشین، EEG داخل جمجمه، EEG پوست سر |
کلمات کلیدی انگلیسی | seizure prediction، convolutional neural network، machine learning، intracranial EEG، scalp EEG |
شناسه دیجیتال – doi |
https://doi.org/10.1016/j.neunet.2018.04.018 |
کد محصول | E10737 |
وضعیت ترجمه مقاله | ترجمه آماده این مقاله موجود نمیباشد. میتوانید از طریق دکمه پایین سفارش دهید. |
دانلود رایگان مقاله | دانلود رایگان مقاله انگلیسی |
سفارش ترجمه این مقاله | سفارش ترجمه این مقاله |
فهرست مطالب مقاله: |
Abstract
۱- Introduction ۲- Proposed method ۳- Results ۴- Discussion ۵- Conclusion References |
بخشی از متن مقاله: |
Abstract Seizure prediction has attracted growing attention as one of the most challenging predictive data analysis efforts to improve the life of patients with drug-resistant epilepsy and tonic seizures. Many outstanding studies have reported great results in providing sensible indirect (warning systems) or direct (interactive neural stimulation) control over refractory seizures, some of which achieved high performance. However, to achieve high sensitivity and a low false prediction rate, many of these studies relied on handcraft feature extraction and/or tailored feature extraction, which is performed for each patient independently. This approach, however, is not generalizable, and requires significant modifications for each new patient within a new dataset. In this article, we apply convolutional neural networks to different intracranial and scalp electroencephalogram (EEG) datasets and propose a generalized retrospective and patient-specific seizure prediction method. We use the short-time Fourier transform on 30-s EEG windows to extract information in both the frequency domain and the time domain. The algorithm automatically generates optimized features for each patient to best classify preictal and interictal segments. The method can be applied to any other patient from any dataset without the need for manual feature extraction. The proposed approach achieves sensitivity of 81.4%, 81.2%, and 75% and a false prediction rate of 0.06/h, 0.16/h, and 0.21/h on the Freiburg Hospital intracranial EEG dataset, the Boston Children’s Hospital–MIT scalp EEG dataset, and the American Epilepsy Society Seizure Prediction Challenge dataset, respectively. Our prediction method is also statistically better than an unspecific random predictor for most of the patients in all three datasets. Introduction Advances in data mining and machine learning in the past few decades have attracted significantly more attention to the application of these techniques in detective and predictive data analytics, especially in health care, medical 5 practices, and biomedical engineering (Kuhlmann et al., 2015; Freestone et al., 2015; Xiao et al., 2017; Bou Assi et al., 2017; Kuhlmann et al., 2017; Freestone et al., 2017; Sinha et al., 2017). While the body of available proven knowledge lacks a convincing and comprehensive understanding of the sources of epileptic seizures, some early studies showed the possibility of predicting seemingly unpredictable 10 seizures (Rogowski et al., 1981; Salant et al., 1998). Along with continuous improvements in recording electroencephalogram (EEG) signals, there have been an increasing number of EEG-based techniques for seizure prediction (Szostak 2 et al., 2017). There have been some articles on seizure prediction using the Freiburg Hospital dataset (University of Freiburg, 2003). For example, the 15 dynamical similarity index, effective correlation dimension, and increments of accumulated energy were used as features (Maiwald et al., 2004). The dynamical similarity index yielded the highest performance, with sensitivity of 42% and false prediction rate (FPR) less than 0.15/h. The mean phase coherence and lag synchronization index of 32-s sliding EEG windows were used as features 20 for seizure prediction (Winterhalder et al., 2006). |