مقاله انگلیسی رایگان در مورد یادگیری عمیق کانولوشن برای محتوا بر اساس بازیابی تصویر (الزویر)
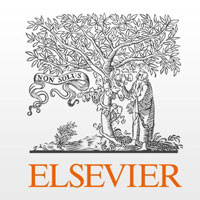
مشخصات مقاله | |
انتشار | مقاله سال ۲۰۱۸ |
تعداد صفحات مقاله انگلیسی | ۱۲ صفحه |
هزینه | دانلود مقاله انگلیسی رایگان میباشد. |
منتشر شده در | نشریه الزویر |
نوع مقاله | ISI |
عنوان انگلیسی مقاله | Deep convolutional learning for Content Based Image Retrieval |
ترجمه عنوان مقاله | یادگیری عمیق کانولوشن برای محتوا بر اساس بازیابی تصویر |
فرمت مقاله انگلیسی | |
رشته های مرتبط | مهندسی کامپیوتر |
گرایش های مرتبط | هوش مصنوعی، مهندسی نرم افزار |
مجله | محاسبات عصبی – Neurocomputing |
دانشگاه | Department of Informatics – Aristotle University of Thessaloniki – Greece |
کلمات کلیدی | بازیابی تصویر بر اساس محتوا، شبکه های عصبی کانولوشن، یادگیری عمیق |
کد محصول | E5646 |
وضعیت ترجمه مقاله | ترجمه آماده این مقاله موجود نمیباشد. میتوانید از طریق دکمه پایین سفارش دهید. |
دانلود رایگان مقاله | دانلود رایگان مقاله انگلیسی |
سفارش ترجمه این مقاله | سفارش ترجمه این مقاله |
بخشی از متن مقاله: |
۱٫ Introduction
Image retrieval is a research area of Information Retrieval [1] of great scientific interest since 1970s. Earlier studies include manual annotation of images using keywords and searching by text [2]. Content Based Image Retrieval (CBIR), [3], has been proposed in 1990s, in order to overcome the difficulties of text-based image retrieval, deriving from the manual annotation of images, that is based on the subjective human perception, and the time and labor requirements of annotation. CBIR refers to the process of obtaining images that are relevant to a query image from a large collection based on their visual content [4]. Given the feature representations of the images to be searched and the query image, the output of the CBIR procedure includes a search in the feature space, in order to retrieve a ranked set of images in terms of similarity (e.g. cosine similarity) to the query representation. A key issue associated with CBIR is to extract meaningful information from raw data in order to eliminate the socalled semantic-gap [5]. The semantic-gap refers to the difference between the low level representations of images and their higher level concepts. While earlier works focus on primitive features that describe the image content such as color, texture, and shape, numerous more recent works have been elaborated on the direction of finding semantically richer image representations. Among the most effective are those that use the Fisher Vector descriptors [6], Vector of Locally Aggregated Descriptors (VLAD) [7,8] or combine bag-of-words models [9] with local descriptors such as ScaleInvariant Feature Transform (SIFT) [10]. Several recent studies introduce Deep Learning algorithms [11] against the shallow aforementioned approaches to a wide range of computer vision tasks, including image retrieval [12–۱۵]. The main reasons behind their success are the availability of large annotated datasets, and the GPUs computational power and affordability. Deep Convolutional Neural Networks (CNN), [16,17], are considered the more efficient Deep Learning architecture for visual information analysis. CNNs comprise of a number of convolutional and subsampling layers with non-linear neural activations, followed by fully connected layers. |