مقاله انگلیسی رایگان در مورد یک روش شبکه عصبی عمیق برای تشخیص خودکار مدولاسیون در OFDM با شاخص مدولاسیون – ۲۰۱۹ IEEE
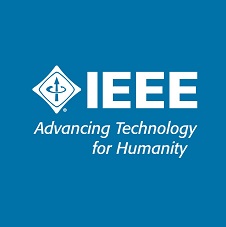
مشخصات مقاله | |
ترجمه عنوان مقاله | یک روش شبکه عصبی عمیق برای تشخیص خودکار مدولاسیون در OFDM با شاخص مدولاسیون |
عنوان انگلیسی مقاله | A Deep Neural Network Method For Automatic Modulation Recognition In OFDM With Index Modulation |
انتشار | مقاله سال ۲۰۱۹ |
تعداد صفحات مقاله انگلیسی | ۵ صفحه |
هزینه | دانلود مقاله انگلیسی رایگان میباشد. |
پایگاه داده | نشریه IEEE |
مقاله بیس | این مقاله بیس نمیباشد |
نوع مقاله | ISI |
فرمت مقاله انگلیسی | |
شناسه ISSN | ۱۰۹۰-۳۰۳۸ |
مدل مفهومی | ندارد |
پرسشنامه | ندارد |
متغیر | ندارد |
رفرنس | دارد |
رشته های مرتبط | کامپیوتر، فناوری اطلاعات |
گرایش های مرتبط | برنامه نویسی کامپیوتر، شبکه های کامپیوتری، هوش مصنوعی، مهندسی نرم افزار |
نوع ارائه مقاله |
کنفرانس |
کنفرانس | ۸۹مین کنفرانس فناوری خودرو – ۸۹th Vehicular Technology Conference |
دانشگاه | Beijing University of Posts and Telecommunications Beijing, P.R.China |
کلمات کلیدی | تشخیص خودکار مدولاسیون (AMR)، یادگیری ماشین، شبکه عصبی عمیق، OFDM، شاخص مدولاسیون |
کلمات کلیدی انگلیسی | automatic modulation recognition (AMR)، machine learning، deep neural network، OFDM، index modulation |
شناسه دیجیتال – doi |
https://doi.org/10.1109/VTCSpring.2019.8746286 |
کد محصول | E13223 |
وضعیت ترجمه مقاله | ترجمه آماده این مقاله موجود نمیباشد. میتوانید از طریق دکمه پایین سفارش دهید. |
دانلود رایگان مقاله | دانلود رایگان مقاله انگلیسی |
سفارش ترجمه این مقاله | سفارش ترجمه این مقاله |
فهرست مطالب مقاله: |
Abstract
I- Introduction II- PROBLEM STATEMENT III- DNN-Based AMR Method IV- Simulation and Results V- Conclusions References |
بخشی از متن مقاله: |
Abstract Automatic modulation recognition (AMR) plays an indispensable role in many fields, such as cognitive radio, spectrum sensing, non-cooperative link adaptation, and other civilian and military fields. OFDM-IM is an innovative OFDMbased scheme which has better bit error performance than classical OFDM scheme especially in high mobility cases. Different from OFDM, the modulation parameters include both M-ary signal constellations and indices of the subcarriers in OFDMIM scheme. In this paper, we studied a deep neural network (DNN) based AMR method in orthogonal frequency division multiplexing with index modulation (OFDM-IM) scheme. INTRODUCTION Orthogonal frequency division multiplexing with index modulation (OFDM-IM) is an innovative transmission scheme which is based on OFDM. OFDM-IM scheme have better performance in high mobility scenarios by exploiting subcarrier indices to carry part of the information [1]. In OFDM-IM scheme, all information bits will be divided into two parts, index selecting bits and constellation modulation bits. In an OFDM-IM frame, all of the subcarriers will be divided into several subblocks. In every subblock, subcarriers will be in one of two states, active or silent. The indices of active subcarriers will be determined by index selecting bits and constellation modulation bits will be systematically mapped into these active subcarriers. AMR is a method to determine modulation parameters from received signal. With the development of modern communications system, the AMR algorithm plays an indispensable role in plenty of civilian and military applications such as spectrum sensing and management, electromagnetic countermeasure, etc. In general, AMR methods can be classified in two classes: likelihood-based (LB) methods and feature-based (FB) methods respectively [2]. The main principle of LB methods is exploiting probabilistic and statistical hypothesis testing. In [3–۷], several likelihood functions were proposed as a LB-AMR method. Though LB methods could have optimal performance, it is hard to implement due to its high computational complexities. Usually, there are two main steps in FB methods, feature extraction and classification. Features for AMR have been studied in many papers including higher order statistics (cumulants [8, 9], statistics [10, 11]), transform domain features (wavelet transforms [12, 13], short-time Fourier transform [14]), etc. Many classification algorithms also have been widely used in AMR such as support vector machine (SVM) [9, 13], minimum distance classifier (MDC) [15], multilayer perceptron (MLP) [16, 17], etc. In lately years, with the rise of machine learning technique, many researchers have made a lot of breakthroughs in wireless communication [18–۲۳]. Machine learning could extend AMR as a powerful classifier. In [21], the author proposed an DNN based AMR method which employs a kind of time-frequency transformation as feature extractor and exploits convolutional neural network (CNN) as classifier. Machine learning could also be an end-to-end AMR method which units feature extraction step and classification step. In [22], the author proposed a recurrent neural network (RNN) based AMR method which takes N timesteps time domain amplitude and phase vector as input. The method also was verified on a standard dataset [24]. In [23], the author proposed a convolution neural network model for AMR which could extract features automatically. With the rapid development of modern communication technology, the new wireless communication scheme brings us new AMR challenge. Different from conventional AMR problem, the modulation parameters in OFDM-IM scheme includes both M-ary signal constellations and indices of the subcarriers in OFDM-IM scheme. Hence, the AMR in OFDMIM scheme is to identify both modulation type M and the number K of active subcarriers in each subblock. In [25], a LB based AMR method was proposed for OFDM-IM scheme and it is the first paper that study on this problem. In this paper, we extend FB based AMR method for OFDM-IM scheme and proposed a practical DNN based method to solve this problem. |