مقاله انگلیسی رایگان در مورد ارزیابی کارایی بر اساس آنالیز پوشش داده ها در زمینه کلان داده ها – الزویر ۲۰۱۸
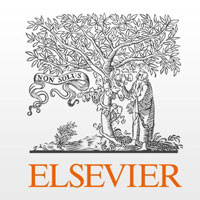
مشخصات مقاله | |
ترجمه عنوان مقاله | ارزیابی کارایی بر اساس آنالیز پوشش داده ها در زمینه کلان داده ها |
عنوان انگلیسی مقاله | Efficiency evaluation based on data envelopment analysis in the big data context |
انتشار | مقاله سال ۲۰۱۸ |
تعداد صفحات مقاله انگلیسی | ۳۱ صفحه |
هزینه | دانلود مقاله انگلیسی رایگان میباشد. |
پایگاه داده | نشریه الزویر |
نوع نگارش مقاله |
مقاله پژوهشی (Research article) |
مقاله بیس | این مقاله بیس نمیباشد |
نمایه (index) | scopus – master journals – JCR |
نوع مقاله | ISI |
فرمت مقاله انگلیسی | |
ایمپکت فاکتور(IF) |
۲٫۹۶۲ در سال ۲۰۱۷ |
شاخص H_index | ۱۲۴ در سال ۲۰۱۸ |
شاخص SJR | ۱٫۹۱۶ در سال ۲۰۱۸ |
رشته های مرتبط | مهندسی صنایع، مدیریت |
گرایش های مرتبط | برنامه ریزی و تحلیل سیستم ها، تحقیق در عملیات |
نوع ارائه مقاله |
ژورنال |
مجله / کنفرانس | کامپیوترها و تحقیق در عملیات – Computers and Operations Research |
دانشگاه | School of Management – University of Science and Technology of China – China |
کلمات کلیدی | تحلیل پوششی داده ها؛ واحد تصمیم گیری؛ محاسبات در مقیاس بزرگ؛ کلان داده |
کلمات کلیدی انگلیسی | Data envelopment analysis; Decision making unit; Large-scale computation; Big data |
شناسه دیجیتال – doi |
http://dx.doi.org/10.1016/j.cor.2017.06.017 |
کد محصول | E10019 |
وضعیت ترجمه مقاله | ترجمه آماده این مقاله موجود نمیباشد. میتوانید از طریق دکمه پایین سفارش دهید. |
دانلود رایگان مقاله | دانلود رایگان مقاله انگلیسی |
سفارش ترجمه این مقاله | سفارش ترجمه این مقاله |
فهرست مطالب مقاله: |
Highlights Abstract Keywords ۱ Introduction ۲ Data envelopment analysis ۳ Algorithms for accelerating the evaluation procedure ۴ Extensions ۵ Case study ۶ Conclusions Acknowledgments References |
بخشی از متن مقاله: |
Abstract
Data envelopment analysis (DEA) is a self-evaluation method which assesses the relative efficiency of a particular decision making unit (DMU) within a group of DMUs. It has been widely applied in real-world scenarios, and traditional DEA models with a limited number of variables and linear constraints can be computed easily. However, DEA using big data involves huge numbers of DMUs, which may increase the computational load to beyond what is practical with traditional DEA methods. In this paper, we propose novel algorithms to accelerate the computation process in the big data environment. Specifically, we firstly use an algorithm to divide the large scale DMUs into small scale and identify all strongly efficient DMUs. If the strongly efficient DMU set is not too large, we can use the efficient DMUs as a sample set to evaluate the efficiency of inefficient DMUs. Otherwise, we can identify two reference points as the sample in the situation of just one input and one output. Furthermore, a variant of the algorithm is presented to handle cases with multiple inputs or multiple outputs, in which some of the strongly efficient DMUs are reselected as a reduced-size sample set to precisely measure the efficiency of inefficient DMUs. Last, we test the proposed methods on simulated data in various scenarios. Introduction Data envelopment analysis (DEA), developed by Charnes et al. [10], is a nonparametric mathematical method used to measure relative efficiency within a group of homogenous decision making units (DMUs), particularly a group with multiple inputs and multiple outputs (see, e.g., [6, 14, 26, 31]). As a nonparametric technique, DEA is not limited by any functional form, and does not require the numerous assumptions that arise from the use of statistical methods for function estimation and efficiency measurement, yet it can evaluate efficiency well (see, e.g., [27, 32, 3, 24]). To date, DEA has been extensively applied in the performance evaluation of hospitals (see, [23, 15]), universities (see, [25, 21]), banks (see, [29, 30]), supply chains (see, [5]), and in many other situations (see, e.g., [19, 28, 33, 35]). DEA measures relative efficiency for a DMU against its peer n-1 DMUs, supposing there are n DMUs in the evaluation system. Traditional DEA models require the solution of a linear programming problem with n+1 variables and m+s constraints, where m and s are the numbers of inputs and outputs, respectively. The traditional DEA models can be solved by using standard linear programming techniques, thus, are theoretically considered computationally easy. However, in practice, the solution time increases significantly for large cases [12]. Emerging in 1980s, the concept of “big data” has become a hot issue in the computer industry and financial businesses. Wu et al. [34] indicated that big data has rapidly expanded in all science and engineering domains, including physical, biological and biomedical sciences. The emergence of the big data paradigm over the past few years, with its five major features (volume, velocity, variety, veracity, and valorization), has created a new set of problems and challenges [36, 8]. For example, Chen et al. [11] highlighted that we can obtain new science, discovery, and insights from the overwhelming amount of web-based, mobile, and sensor-generated data arriving at a terabyte and even exabyte scale. Michael and Miller [22] indicated that the development of big data could bring comprehensive analyses to support the development of improved policy regime-based systems. In the DEA field, big data has brought many problems for researchers. For example, the larger scale number of DMUs in the big data context is the biggest issue since it may take an impractical amount of time to finish the evaluation of all DMU efficiencies. Therefore, methods for reducing solution time for DEA problems are practically beneficial, especially in the big data environment. |