مقاله انگلیسی رایگان در مورد یادگیری الکترونیکی مبتنی بر خودسازماندهی منابع یادگیری – الزویر ۲۰۱۸
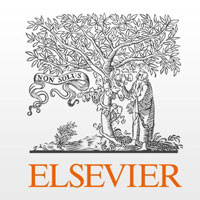
مشخصات مقاله | |
ترجمه عنوان مقاله | یک رویکرد پیشنهاد یادگیری الکترونیکی مبتنی بر خودسازماندهی منابع یادگیری |
عنوان انگلیسی مقاله | An e-learning recommendation approach based on the self-organization of learning resource |
انتشار | مقاله سال ۲۰۱۸ |
تعداد صفحات مقاله انگلیسی | ۳۹ صفحه |
هزینه | دانلود مقاله انگلیسی رایگان میباشد. |
پایگاه داده | نشریه الزویر |
نوع نگارش مقاله |
مقاله پژوهشی (Research article) |
مقاله بیس | این مقاله بیس نمیباشد |
نمایه (index) | scopus – master journals – JCR |
نوع مقاله | ISI |
فرمت مقاله انگلیسی | |
ایمپکت فاکتور(IF) |
۴٫۳۹۶ در سال ۲۰۱۷ |
شاخص H_index | ۸۲ در سال ۲۰۱۸ |
شاخص SJR | ۱٫۳۷۸ در سال ۲۰۱۸ |
رشته های مرتبط | علوم تربیتی |
گرایش های مرتبط | تکنولوژی اموزشی |
نوع ارائه مقاله |
ژورنال |
مجله / کنفرانس | سیستم های مبتنی بر دانش – Knowledge-Based Systems |
دانشگاه | Beijing Institute of Technology – Beijing – China |
کلمات کلیدی | سیستم پیشنهادی شخصی، یادگیری الکترونیکی، خودسازمانی، تنوع، سازگاری |
کلمات کلیدی انگلیسی | personalized recommender system, e-learning, self-organization, diversity, adaptability |
شناسه دیجیتال – doi |
https://doi.org/10.1016/j.knosys.2018.06.014 |
کد محصول | E9859 |
وضعیت ترجمه مقاله | ترجمه آماده این مقاله موجود نمیباشد. میتوانید از طریق دکمه پایین سفارش دهید. |
دانلود رایگان مقاله | دانلود رایگان مقاله انگلیسی |
سفارش ترجمه این مقاله | سفارش ترجمه این مقاله |
فهرست مطالب مقاله: |
Abstract Keywords ۱ Introduction ۲ Related works ۳ Preliminaries ۴ LO self-organization based recommendation approach ۵ Experiment setup ۶ Results and discussions ۷ Conclusions and future work Acknowledgment References |
بخشی از متن مقاله: |
Abstract
In e-learning, most content-based (CB) recommender systems provide recommendations depending on matching rules between learners and learning objects (LOs). Such learner-oriented approaches are limited when it comes to detecting learners’ changes, furthermore, the recommendations show low adaptability and diversity. In this study, in order to improve the adaptability and diversity of recommendations, we incorporate an LO-oriented recommendation mechanism to learner-oriented recommender systems, and propose an LO self-organization based recommendation approach (Self). LO self-organization means LO interacts with each other in a spontaneous and autonomous way. Such self-organization behavior is conducive to generating a stable LO structure through information propagation. The proposed approach works as follows: firstly, LOs are simulated as intelligent entities using the self-organization theory. LOs can receive information, transmit information, as well as move. Secondly, an environment perception module is designed. This module can capture and perceive learner’s preference drifts by analyzing LOs’ self-organization behaviors. Finally, according to learners’ explicit requirements and implicit preference drifts, recommendations are generated through LOs’ self-organization behaviors. Based on application to real-life learning processes, the ample experimental results demonstrate the high adaptability, diversity, and personalization of the recommendations. Introduction E-learning recommender systems aim to recommend a sequence of items to learners, that is, to suggest the most efficient or effective paths through a plethora of learning resources to achieve a certain competence [1, 2]. However, over specification and excessive searching in e-learning recommender systems result 5 in information overload. Learners do not have enough time to deal with these massive recommendations. In addition, the adaptability and diversity of recommendations are desirable in e-learning recommender systems, because learners’ preferences and abilities keep changing, and also because the functionality of some learning resources for active learners keeps changing. The diverse and adaptive recommendations should be presented to motivate learning potential of learners and ensure a long-term learning experience. 10 In this study, we aim to improve the adaptability and diversity of content-based (CB) recommendations. Generally, learning resources are filtered in three ways: CB, collaborative filtering (CF), and hybrid filtering (HF) [3, 4]. CF recommender systems compute the similarity computation between learners based on their rating and then make predictions[5, 6]. CF methods are known to suffer from the rating sparsity problem which occurs when the users or items have insufficient rating records [7]. In e-learning, 15 the rating information is extremely sparse. The main reasons for this are a lack of motivation for learners to rate, a lack of scoring mechanisms, and the scheduled but limited learning time of learners [1]. The high data sparsity makes it difficult to apply CF techniques. CB recommender systems recommend relevant learning contents which are highly matched to learners’ learning goals and preferences. In addition, CB recommender systems do not usually suffer from the first-rater problem, therefore, CB recommendation 20 approaches are the only ones capable of recommending items not previously rated by any learner [8]. The learner’s ability, goal, mood, and cognitive style are often used as criteria in CB recommendation systems [8, 9]. |