مقاله انگلیسی رایگان در مورد تعبیه متریک تمایزی نمایی در یادگیری عمیق – الزویر ۲۰۱۸
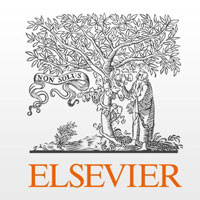
مشخصات مقاله | |
انتشار | مقاله سال ۲۰۱۸ |
تعداد صفحات مقاله انگلیسی | ۳۴ صفحه |
هزینه | دانلود مقاله انگلیسی رایگان میباشد. |
منتشر شده در | نشریه الزویر |
نوع مقاله | ISI |
عنوان انگلیسی مقاله | Exponential Discriminative Metric Embedding in Deep Learning |
ترجمه عنوان مقاله | تعبیه متریک تمایزی نمایی در یادگیری عمیق |
فرمت مقاله انگلیسی | |
رشته های مرتبط | مهندسی کامپیوتر |
گرایش های مرتبط | هوش مصنوعی |
مجله | محاسبات عصبی – Neuro computing |
دانشگاه | Center for Combinatorics – Nankai University – China |
کلمات کلیدی | یادگیری متریک عمیق، تشخیص موضوع، تایید سطح، فشرده سازی داخل رده، تفکیک بین رده |
کلمات کلیدی انگلیسی | Deep metric learning, Object recognition, Face verification, Intra-class compactness, Inter-class separability |
کد محصول | E6031 |
وضعیت ترجمه مقاله | ترجمه آماده این مقاله موجود نمیباشد. میتوانید از طریق دکمه پایین سفارش دهید. |
دانلود رایگان مقاله | دانلود رایگان مقاله انگلیسی |
سفارش ترجمه این مقاله | سفارش ترجمه این مقاله |
بخشی از متن مقاله: |
۱٫ Introduction
Recently, Convolutional Neural Networks (CNNs) are continuously setting new records in classification aspect, such as object recognition [1, 2, 3, 4], scene recognition [5, 6], face recognition [7, 8, 9, 10, 11, 12], age estimation [13, 14] and so on. Facing the more and more complex data, the deeper and wider CNNs tend to obtain better accuracies. Meanwhile, many troubles will show up, such as gradient saturating, model overfitting, parameter augmentation, etc. To solve the first problem, some non-linear activations [15, 16, 17] have been proposed. Considerable efforts have been made to reduce model overfitting, such as data augmentation [1, 18], dropout [19, 1], regularization [15, 20]. Besides, some model compressing methods [21, 22] have largely reduced the computing complexity of original models, with the performance improved simultaneously. In general object recognition, scene recognition and age estimation, the identities of the possible testing samples are within the training set. So the training and testing sets have the same object classes but not the same images. In this case, softmax classifier is often used to designate a label to the input. For face recognition, the deeply learned features need to be not only separable but also discriminative. It can be roughly divided into two aspects, namely face identification and face verification. The former is the same as object recognition, the training and testing sets have the same face identities, aims at classifying an input image into a large number of identity classes. Face verification is to classify a pair of images as belonging to the same identity or not (i.e. binary classification). Since it is impractical to precollect enough number of all the possible testing identities for training, face verification is becoming the mainstream in this field. As clarified by DeepID series [9, 23, 10]: classifying all the identities simultaneously instead of binary classifiers for training can make the learned features more discriminative between different classes. So we decide to use the joint supervision of softmax classifier and metric loss function to train and the verification signal of feature similarity discriminant to test as shown in Section 4.3. Fig. 1 illustrates the general face recognition pipeline, which maps the input images to the discriminative deep features progressively, then to the predicted labels. |