مقاله انگلیسی رایگان در مورد روش پیش بینی مانور بر اساس شبکه بیزی دینامیکی – IEEE 2018
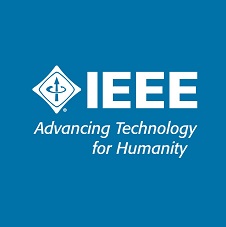
مشخصات مقاله | |
ترجمه عنوان مقاله | یک روش پیش بینی مانور بر اساس شبکه بیزی دینامیکی در سناریوهای بزرگراه |
عنوان انگلیسی مقاله | A maneuver-prediction method based on dynamic bayesian network in highway scenarios |
انتشار | مقاله سال ۲۰۱۸ |
تعداد صفحات مقاله انگلیسی | ۶ صفحه |
هزینه | دانلود مقاله انگلیسی رایگان میباشد. |
منتشر شده در | نشریه IEEE |
نوع مقاله | ISI |
فرمت مقاله انگلیسی | |
رشته های مرتبط | مهندسی کامپیوتر، فناوری اطلاعات |
گرایش های مرتبط | هوش مصنوعی، شبکه های کامپیوتری |
مجله | کنفرانس کنترل و تصمیم گیری در چین – Chinese Control And Decision Conference |
دانشگاه | National University of Defense Technology – Changsha – P.R.China |
کلمات کلیدی | رانندگی خودکار، پیش بینی مانور، استخراج ویژگی، شبکه بیزی دینامیکی |
کلمات کلیدی انگلیسی | Autonomous driving, maneuver prediction, feature extraction, Dynamic Bayesian Network |
شناسه دیجیتال – doi |
https://doi.org/10.1109/CCDC.2018.8407710 |
کد محصول | E8934 |
وضعیت ترجمه مقاله | ترجمه آماده این مقاله موجود نمیباشد. میتوانید از طریق دکمه پایین سفارش دهید. |
دانلود رایگان مقاله | دانلود رایگان مقاله انگلیسی |
سفارش ترجمه این مقاله | سفارش ترجمه این مقاله |
بخشی از متن مقاله: |
۱ INTRODUCTION
Many traffic accidents happen in complex traffic environments. The accurate motion prediction for surrounding vehicles can enhance driving safety in these complex environments. As to autonomous driving, the accurate motion prediction for surrounding vehicles, which are also called as participant vehicles (PVs), will contribute to make safer decisions and plan smoother trajectories. Motion prediction in autonomous driving provides a human-like way of driving that can predict maneuvers of surrounding vehicles. Similarly, accurate motion prediction for PVs in Advanced Driver Assistance Systems (ADAS) is also useful for human drivers. In recent years, a large number of new techniques have been proposed to predict the motion of participant vehicles. In [1], the approaches of motion prediction are classified into three categories, namely physics-based approaches, maneuver-based approaches and interactionaware approaches. However, many researches always combine interaction-aware approaches with the other two approaches for more accurate motion prediction results [2]. Moreover, the motion prediction approaches are classified into four categories in [3] according to the prediction number of future behaviors. Different from these two classification methods, we classify the motion prediction approaches into two categories: 1) trajectory prediction and 2) maneuver prediction. Trajectory prediction deals with the prediction in the short term, whereas the maneuver prediction predicts the motion in the long term. Trajectory prediction predicts the trajectories of PVs. Basically, these approaches use current states of PVs and the kinematic model of vehicles. For instance, continu ous Bayes filters (e.g., Kalman Filter) are applied in [4, 5]. Besides, probabilistic methods [2] are also used in trajectory prediction to cope with uncertainty and inaccuracy. In [6, 7], an offline learning trajectory set learned by Gaussian process and an improved RRT are combined to predict trajectories. However, driving trajectories are related to longterm maneuvers, mere trajectory prediction is not accurate in long term. Maneuver prediction gradually receives more attention in recent years. The maneuver prediction is usually more reliable in the long term. For instance, the approach in [8] can detect a lane-change maneuver averagely 0.6 s earlier than an adaptive cruise control (ACC) system. An objectoriented Bayesian network (OOBN) is proposed to predict maneuvers of PVs in [8]. The naive Bayesian method is extended in [9] for lane-change maneuver prediction. Sivaraman and Trivedi [10] introduce a dynamic probability drivability map (DPDM) for a driver assistant system, which can predict maneuvers of other vehicles. In [11], a method that combines the field map and a Bayesian network is presented, focusing on the interaction-aware maneuver prediction. Recently, a Support Vector Machine (SVM) method is used to predict lane-change maneuvers in [12] with consideration of traffic interactions. In order to consider interactions among vehicles, Dynamic Bayesian Networks (DBNs) are used in the maneuver prediction. Inspired by [13], this paper presents a novel Dynamic Bayesian Network for the maneuver prediction (DBMP) in highway scenarios. The advantages of our DBMP include two aspects. 1) DBMP predicts maneuvers of PVs based on multi-frame features, the road structure, and interactions among PVs together in a unified framework. 2) DBMP combines the driving experience of humans with machine learning algorithm. |