مقاله انگلیسی رایگان در مورد همگرایی غیر یکنواخت الگوریتم های یادگیری آنلاین پرسپترون – الزویر ۲۰۱۸
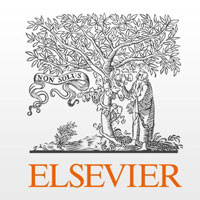
مشخصات مقاله | |
انتشار | مقاله سال ۲۰۱۸ |
تعداد صفحات مقاله انگلیسی | ۶ صفحه |
هزینه | دانلود مقاله انگلیسی رایگان میباشد. |
منتشر شده در | نشریه الزویر |
نوع مقاله | ISI |
عنوان انگلیسی مقاله | Non-monotonic convergence of online learning algorithms for perceptrons with noisy teacher |
ترجمه عنوان مقاله | همگرایی غیر یکنواخت الگوریتم های یادگیری آنلاین برای پرسپترون با مدرس پر سر و صدا |
فرمت مقاله انگلیسی | |
رشته های مرتبط | کامپیوتر و فناوری اطلاعات |
گرایش های مرتبط | مهندسی الگوریتم ها و محاسبات، هوش مصنوعی، شبکه های کامپیوتری |
مجله | شبکه های عصبی – Neural Networks |
دانشگاه | Nara Institute of Science and Technology – Ikoma – Nara – Japan |
کلمات کلیدی | منحنی یادگیری، پرسپترون، یادگیری آنلاین، مکانیک آماری، تجزیه و تحلیل همپوشانی |
کلمات کلیدی انگلیسی | Learning curve, Perceptron, Online learning, Statistical mechanics, Asymptotic analysis |
کد محصول | E6241 |
وضعیت ترجمه مقاله | ترجمه آماده این مقاله موجود نمیباشد. میتوانید از طریق دکمه پایین سفارش دهید. |
دانلود رایگان مقاله | دانلود رایگان مقاله انگلیسی |
سفارش ترجمه این مقاله | سفارش ترجمه این مقاله |
بخشی از متن مقاله: |
۱٫ Introduction
Statistical mechanical methods can apply to problems in information science such as neural networks (Nishimori, 2001), communication theory (Tanaka, 2002), and adaptive filters (Miyoshi & Kajikawa, 2013). One successful application is the analyses of the perceptron learning algorithm (Biehl & Schwarze, 1992; Rosenblatt, 1961) and its variations (Hara & Okada, 2004; Inoue & Nishimori, 1997; Miyoshi, Hara,& Okada, 2005; Miyoshi& Okada, 2006a, b; Uezu, Miyoshi, Izuo, & Okada, 2007). The perceptron learning is an online learning algorithm where the student updates its weight vector of a linear dichotomy according to the teacher’s signal (Rosenblatt, 1961). Biehl and Schwarze (1992) introduced the statistical mechanics to the analysis of the perceptron learning and Inoue and Nishimori (1997) applied the method to the AdaTron learning in unlearnable cases. Hara and Okada (2004) discussed the perceptron learning with a margin and Miyoshi and his colleagues extended the analysis to the ensemble learning and/or noisy cases (Miyoshi et al., 2005; Miyoshi & Okada, 2006a, b; Uezu et al., 2007). In this paper, we consider the case where the teacher has noise in its output while the student does not. In this case, the learning curve, which is defined as the average prediction error, is not monotonically decreasing but has an overshoot, differently from other cases analyzed so far (Ikeda, Hanzawa, & Miyoshi, 2013). Although an analysis for this problem was partially given by some of the authors (Ikeda et al., 2013), some part was given not theoretically but numerically. This paper gives a theoretically rigorous and complete analysis above. In addition, we extend the analysis to other online algorithms for perceptrons, that is, the AdaTron learning and the Hebbian learning. Our analysis consisted of three steps. In the first step, we applied the statistical mechanical method to our problem, i.e., we introduced three order parameters assuming the thermodynamic limit, and derived a system of differential equations for the three algorithms. In the second step, we calculated the ensemble averages that appeared in the differential equations for each algorithm using Gaussian approximations. Note this had not been derived analytically yet in Ikeda et al. (2013). In the last step, we applied an asymptotic analysis to our dynamical system, i.e., we linearized the equations around their convergence point and analyzed their behaviors by the eigenvalues and eigenvectors of the state-transition matrix a.k.a. the system matrix. The three steps elucidated how and why the overshoot phenomenon occurs. The remainder of this paper is organized as follows. Section 2 formulates the problem we treated. Sections 3–۵ are devoted to the three steps, that is, statistical mechanical analysis, the calculation of the ensemble averages and the asymptotic analysis of the system, respectively. We conclude the paper in Section 6. |