مقاله انگلیسی رایگان در مورد آنالیز داده های رسانه اجتماعی برای بهبود مدیریت زنجیره تامین – الزویر ۲۰۱۸
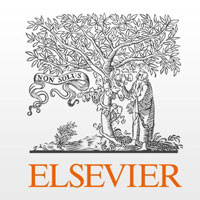
مشخصات مقاله | |
ترجمه عنوان مقاله | آنالیز داده های رسانه های اجتماعی برای بهبود مدیریت زنجیره تامین در صنایع غذایی |
عنوان انگلیسی مقاله | Social media data analytics to improve supply chain management in food industries |
انتشار | مقاله سال ۲۰۱۸ |
تعداد صفحات مقاله انگلیسی | ۱۸ صفحه |
هزینه | دانلود مقاله انگلیسی رایگان میباشد. |
پایگاه داده | نشریه الزویر |
نوع نگارش مقاله |
مقاله پژوهشی (Research article) |
مقاله بیس | این مقاله بیس نمیباشد |
نمایه (index) | scopus – master journals – JCR |
نوع مقاله | ISI |
فرمت مقاله انگلیسی | |
ایمپکت فاکتور(IF) |
۳٫۲۸۹ در سال ۲۰۱۷ |
شاخص H_index | ۸۵ در سال ۲۰۱۸ |
شاخص SJR | ۱٫۹۰۱ در سال ۲۰۱۸ |
رشته های مرتبط | مهندسی صنایع، فناوری اطلاعات |
گرایش های مرتبط | لجستیک و زنجیره تامین، اینترنت و شبکه های گسترده |
نوع ارائه مقاله |
ژورنال |
مجله / کنفرانس | تحقیقات حمل و نقل – Transportation Research Part E |
دانشگاه | Alliance Manchester Business School – University of Manchester – UK |
کلمات کلیدی | زنجیره تامین گوشت گاو، اطلاعات توییتر،، تحلیل احساسات |
کلمات کلیدی انگلیسی | Beef supply chain, Twitter data, Sentiment analysis |
شناسه دیجیتال – doi |
http://dx.doi.org/10.1016/j.tre.2017.05.008 |
کد محصول | E10066 |
وضعیت ترجمه مقاله | ترجمه آماده این مقاله موجود نمیباشد. میتوانید از طریق دکمه پایین سفارش دهید. |
دانلود رایگان مقاله | دانلود رایگان مقاله انگلیسی |
سفارش ترجمه این مقاله | سفارش ترجمه این مقاله |
فهرست مطالب مقاله: |
Highlights Abstract Keywords ۱ Introduction ۲ Related work ۳ Twitter data analysis process ۴ Case study and Twitter data analysis ۵ Identification of issues affecting consumer satisfaction and their mitigation within the supply chain ۶ Managerial implications ۷ Conclusions Acknowledgement References |
بخشی از متن مقاله: |
abstract
This paper proposes a big-data analytics-based approach that considers social media (Twitter) data for the identification of supply chain management issues in food industries. In particular, the proposed approach includes text analysis using a support vector machine (SVM) and hierarchical clustering with multiscale bootstrap resampling. The result of this approach included a cluster of words which could inform supply-chain (SC) decision makers about customer feedback and issues in the flow/quality of food products. A case study in the beef supply chain was analysed using the proposed approach, where three weeks of data from Twitter were used. Introduction In the modern era, food is a crucial commodity for consumers, as it has a direct impact on their health (Caplan, 2013; Swaminathan, 2015; Tarasuk et al., 2015). The food supply chain is more complicated than the manufacturing and other conventional supply chains, owing to the perishable nature of food products (La Scalia et al., 2015; Handayati et al., 2015). Food retailers aim to adjust their supply chain to become consumer centric (a supply chain designed as per the requirements of end consumers by addressing organisational, strategic, technology, process, and metrics factors) by taking into account various methods, including market surveys, market research, interviews, and offering the opportunity to consumers to provide feedback within the retailer store. However, food retailers are not able to attract large audiences by following these procedures; thus, their data sample is small. Any decisions made based on a smaller sample of customer feedback are prone to be ineffective. With the advent of online social media, there is substantial amount of consumer information available on Twitter, which reflects the true opinion of customers (Liang and Dai, 2013; Katal et al., 2013). Effective analysis of this information can provide interesting insight into consumer sentiments and behaviours with respect to one or more specific issues. Using social media data, a retailer can capture a real-time overview of consumer reactions regarding an episodic event. Social media data are relatively inexpensive, and can be very effective in gathering the opinions of large and diverse audiences (Liang and Dai, 2013; Katal et al., 2013). Using different information techniques, business organisations can collect social media data in real time, and can use it for the development of future strategies. However, social media data are qualitative and unstructured in nature, and are often large in volume, variety, and velocity (He et al., 2013; Hashem et al., 2015; Zikopoulos and Eaton, 2011). At times, it is difficult to handle them using the traditional operation and management tools and techniques for business purposes. In the past, social media analytics have been implemented in various supply chain problems, predominantly in manufacturing supply chains. The research on the application of social media analytics in the domain of the food supply chain is in its primitive stage. In the present work, an attempt has been made to use social media data in the domain of the food supply chain to transform it into a consumer-centric supply chain. The results from the analysis have been linked with all the segments of the supply chain to improve customer satisfaction. For instance, the issues faced by consumers of beef products, such as discoloration, presence of foreign bodies, extra fat, and hard texture, have been linked to their root causes in the upstream of the supply chain. First, data were extracted from Twitter (via the Twitter streaming application programming interface (API)) using relevant keywords related to consumer opinion on different food products. Thereafter, pre-processing and text mining was performed to investigate the positive and negative sentiments of tweets, using a support vector machine (SVM). Hierarchical clustering of tweets from different geographical locations (world, UK, Australia, and the USA) using multiscale bootstrap resampling was performed. Furthermore, root causes of issues affecting consumer satisfaction were identified and linked with various segments of the supply chain to render it more efficient. Finally, recommendations for a consumer-centric supply chain were prescribed. |